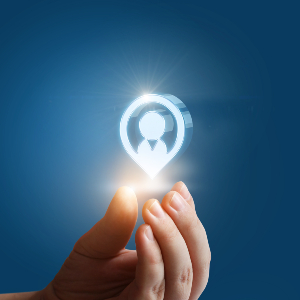
Upland BlueVenn examined 4000 consumers and 500 marketers across the US and UK in 2021, and found out that an average consumer uses 20 marketing channels while interacting with a brand. Furthermore, 84% of marketers agreed that one of the biggest challenges they face is unifying customer data and finding individual customers, since they have multiple digital identities. On the other hand, 55% of consumers notably stated that a personalized shopping experience is critical while making a buying decision.
These statistics highlight the importance of leveraging data to understand your marketing audience: who they are, what interests them, and why they would buy from you. But most marketers mention that at least 42.5% of their data is not being utilized to answer such questions. And the number one reason behind this is poor data quality. Due to data quality issues, marketers do not trust the data they have, and would rather risk missing out on big market opportunities than use incorrect information.
Where is Customer Data Coming From?
Before we dive into specifics of handling and unifying customer data, we must first identify the sources that generate data related to leads, prospects, or customers. As mentioned above, a consumer uses about 20 marketing channels while interacting with a brand. Let’s take a look at what these channels are:
- Web browser (laptop/desktop PC)
- In-store
- Mobile app
- TV
- Mobile web browser
- YouTube
- Over the phone
- Newspaper (print or online)
- Text message
- Internet connected speaker (e.g., Alexa)
- Radio
- Chatbots
- Catalogue
- TikTok
- Post
Factors Affecting the Channel Preferences of Consumers
1. Verticals/industries
The usage of these marketing channels listed above may differ depending on what a consumer is searching to buy. For example, while buying clothes or homeware, consumers are more likely to make a purchase physically in a store. But while shopping for insurance products, they’d rather finalize the decision over a phone call.
2. Consumer location
Channel selection also varies depending on the country/state a consumer is located in. For example, UK consumers prefer shopping online for clothes and homeware using web browsers, while US consumers would rather shop in-store.
Handling the Surge of Data
No matter what channels consumers prefer while making a buying decision, one thing is for sure: a high influx of information is being generated by consumers, and brands must employ a systematic process for handling this data, and converting it into a usable format.
A customer’s data may contain – but is not limited to:
- Shopping behavior and preferences
- Buying journey (ordered list of all touchpoints leading to a purchase)
- Personal information (name, gender, address, contact information, etc.)
- Device information (IP data or other information that uniquely identifies the devices used by a consumer)
- Digital information (email addresses, social profiles, website visits, etc.)
Let’s not forget that this data is being captured across disparate sources, and probably being stored in different formats, sizes, and data types. This brings us to the burning topic of unifying customer data and removing duplicates to uncover underlying digital identities.
The Process of Unifying Customer Data
Unifying customer data is a simple step-by-step process, but depending on the nature of your data, you may require expert consultation to get the most out of your data.
A brief explanation of the steps involved is given below:
1. Bringing data together
The first step of the process is to identify all data sources that track and store customer information. These sources can be Excel sheets, relational or non-relational databases, or third-party applications. You need to gather the data scattered across sources at one place before any processing can begin. This can be done manually, or by using a central data management hub that connects and pulls data from all these places.
You might encounter differences in data columns since each dataset has its own set of data attributes. To resolve this, you will need to import selected columns as well as map columns that contain the same information.
2. Uncovering hidden details of data
Once you have the data together, the next step is to profile data and build a quick assessment of the data at hand. This will uncover hidden details of your data as well as highlight potential data cleansing opportunities.
A data profile generally includes the percentage of customer records that have:
- Missing or incomplete values (such as missing phone numbers),
- Invalid patterns (such as email addresses not following valid patterns like abs@xyz.com),
- Incorrect data types (such as phone numbers being saved as characters, rather than digits),
- Incorrect formats (such as date being saved as DD-MM-YYYY, rather than MM-DD-YYYY)
- Unusual sizes (such as first names running long having 50+ characters),
and other such descriptive statistics.
3. Standardizing dataset
This step includes taking corrective measures for the discrepancies uncovered in the data profile report, such as filling in missing information, validating patterns, transforming data values, and so on.
While standardizing the dataset, you also need to prepare data for the next step, which is data matching. This can involve parsing one column into multiple columns to enable better match results. For example, parsing the Address column into Street Number, Street Name, Area, City, State, and Zip Code.
4. Matching data to detect duplicates
You are now ready to find and link records that belong to the same individual. To achieve that, start by finding out the uniquely identifying attributes of your dataset. For example, if your website tracking tool captures the user’s IP address, you can match IP addresses to find out which records are generated from the same consumer.
In absence of such identifiers, you may have to use complex fuzzy matching techniques that compare two non-exact data values and assess the likelihood of them belonging to the same individual. For example, you can select a combination of attributes, and perform:
- Fuzzy match on customer’s Name and Email Address, and
- Exact match on their Phone Number.
Since records may be missing data values for some of these attributes, matching on a combination of fields usually results in more accurate matches.
5. Deduplicating and merging data records
In the final step, you can review the results generated by match algorithms and validate that the records are correctly labelled as matches, and there are no false positives and negatives in the results.
Once done, now it’s time to merge records to ensure that all data is utilized or exported in the final master record. To achieve that, you can select master records, and also decide what happens to the data stored in the duplicate records – whether you want to discard them, or overwrite/append data into the master record.
When you have deduplicated and merged data records, your dataset is now ready to be exported or loaded to any database of choice.
Enabling Personalized Customer Experiences Using Unified Dataset
And there you have it, a unified, 360 view of your consumers. A profiled, cleaned, standardized, and deduplicated dataset is the biggest asset for designing personalized experiences for your marketing audience. It clearly helps you to understand who your customers are, how they feel, and what they are looking for. If you design customer experiences based on reliable data insights, you will definitely be more successful in winning customers as compared to the ones that shoot darts in the dark. This is why employing an end-to-end data quality management strategy for your marketing datasets can help you to get the most out of your big data, efficiently and effectively .