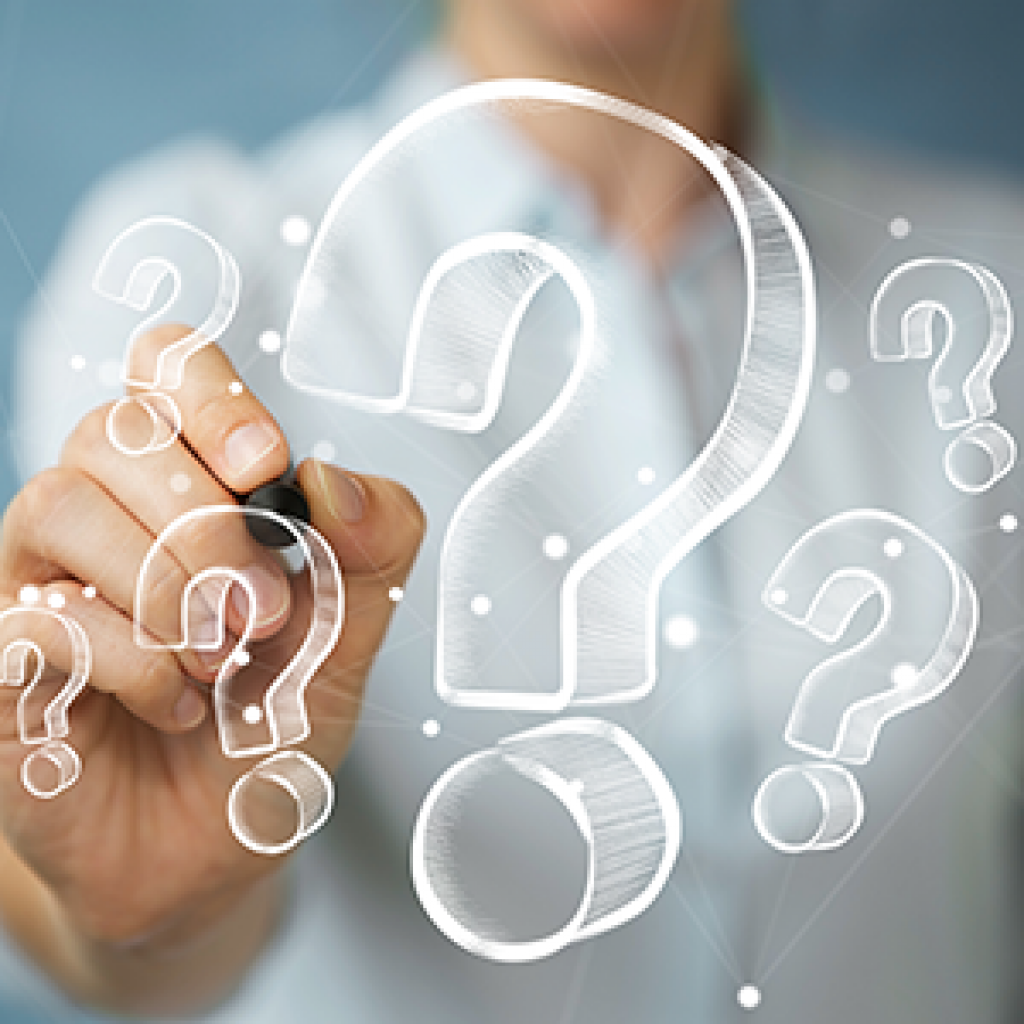
Data quality is measured across dimensions, but why? Data quality metrics exist to support the business. The value of a data quality program resides in the ability to take action to improve data to make it more correct and therefore more valuable. The shorter the amount of time between the discovery of the data quality issue and the resolution, the better. Asking very targeted questions — in the form of data quality rules, that fall within one data quality dimension — shortens the response time of the rule and, therefore, shortens the time to resolution.
Danette McGilvray defines data quality dimensions as “characteristics, aspects, or features of data” in her well-known book “Executing Data Quality Projects: Ten Steps to Quality Data and Trusted Information.” Utilizing one and only one dimension enforces the granularity of the data quality rule, which results in a quick response time. Let’s walk through a true-to-life example to understand.
Can you recall the last time you went shopping for new clothes? You find yourself in a fitting room and then hear the inevitable knock on the door followed by a “How are you doing in there? Can I get you anything?” Let’s walk through a couple of scenarios that may follow.
Most ideally, I give a quick “I’m doing just fine, thanks!” I am checking myself out in the mirror in a fabulous new shirt. In these instances, the item meets my standards and I am satisfied. Such is the case with data quality rules. Often, we focus on poor data quality (because this is our opportunity area to support the business) but, in truth, a lot of us have some great data that the business is already using as a valuable asset. Win!
The next most ideal return I would shout back to a salesperson is something like, “Do you have this same shirt in a smaller size?” or “I like the fit of this shirt, but this color doesn’t work for me. Do you have the same shirt in green?” In those situations, the very targeted feedback clarifies exactly which characteristic of the shirt is not meeting standards, which then provides an opportunity for an immediate response. In this instance, the resolution is also possible and quick, resulting in a more fitting product (no pun intended).
In this scenario, the “How are you doing in there?” is incredibly open-ended. I could have said anything in response. A fully-fledged data governance framework would have instead started with a clear critical data element (CDE) definition to ensure we measure the right piece of clothing, followed by a series of standards like “shirt must be green” and “shirt must be size small.” From there, very targeted data quality rules are easy to establish. Is the shirt green? Is the shirt size small? The measurement of the rules would give a clear indication if the standards are met, with no additional feedback required.
Of course, the clear, concise standards do not automatically suggest that the rule you pose can be answered in the positive. You could just as easily receive the response that the color you preferred is out of stock. While not the ideal you hoped for, the response is still quick and clear. Similarly, there are legitimate reasons why a very specific targeted data quality rule with failing results cannot be remediated right away (time, money, priority). While this can be hard to hear, the information is valuable. Better to have the information in hand to be able to correctly prioritize and strategize.
To be sure, the well-articulated data quality rule results in a quick response, but not necessarily an easy remediation. Something like, “I like the look of the shirt, but I wanted something to keep me warm in winter. Do you offer this shirt in a thicker fabric?” may or may not be something the store offers. Here more than ever, is it correctly identifying and communicating the characteristic, aspect, or feature of the shirt that is not meeting your standards, which will provide the best response and will help to determine the path forward. Perhaps the store offers the ability to create a custom shirt and you can work with them to create an alternative that meets your needs. The resolution timeframe is clearly longer here. If instead the shop does not offer this kind of customization, again the clarity will help you to correctly strategize. Perhaps you will move on to a different store whose products will better align with your needs. These may not be ideal options, but at least you have the information you need to determine a path forward.
The less pointed the rule, the worse the outcome. Creating a data quality rule without a specific dimension is akin to responding to that salesperson’s initial inquiry with “I don’t like this item; can you help me find something different?” I would be giving the salesperson no information. They would be taking a shot in the dark at best, or they’d have to follow up with questions: “Are you looking for a specific type of clothing?” “Are you looking for an outfit for a particular event or everyday wear?” “What’s your price point?” These are great questions to hone in on the need, but it is entirely unlikely they would lead to a quick resolution. This is akin to a data quality rule that leads to root cause analysis instead of resolution. A rule like that may feel productive and progress in the right direction, but is it really?
I don’t mean to discourage — quite the opposite. If your metrics are currently providing information, but they are not dimension-specific, you have a great opportunity to increase the value of your rules library by creating similar dimension-specific rules. Once you have a few rules measuring the same data across dimensions, you’ll be able to use the output in tandem to truly understand the data landscape.