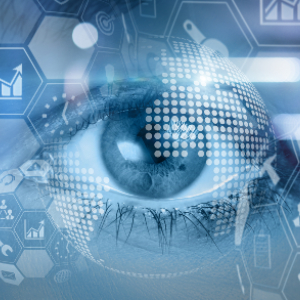
Artificial Intelligence (AI), Machine Learning (ML) and Large Language Models (LLM) have turned the world on its head. From finance to manufacturing to pharmaceuticals to retail, every industry is jumping on the AI/ML bandwagon. And for good reason. AI/ML has the ability to improve efficiency, drive automation, and shorten delivery cycles. AI/ML applications can absorb and report on massive amounts of data that would take the average analyst days if not weeks or months to sort through and analyze. Overall, AI/ML will help the decision-makers make better decisions in shorter periods of time.
But, like any powerful capability, one must proceed with caution. If incorrectly implemented, AI/ML can take on a life of its own— spewing out inconsistent information, exposing unacceptable biases and drawing unethical conclusions. The AI/ML space has created an interesting conundrum: many organizations are fearful of jumping into the AI/ML pool (becoming the risky “early adopters”) while at the same time, are fearful of NOT jumping into the AI/ML pool (experiencing FOMO — Fear of Missing Out).
So, how to proceed? How can you maximize the benefits of AI/ML while minimizing the pitfalls and risks? I believe a big chunk of the answer is out there already — found in the deployment of “good old-fashioned” data management.
Data Management is More Than Data Governance
Now your first reaction might be, “Isn’t the title of this article about the importance of data governance when implementing AI/ML?” Why are you saying it’s about data management? Well… because it is! I referred to data governance in the title to lure you in! Why? Because too often, I see organizations assume that the solution to managing their AI/ML programs is through data governance. The truth is, they’re not wrong, but they’re only partially right. Data governance is actually just a component of data management. To fully embrace and take advantage of your AI/ML program, to leverage its capabilities while mitigating risk, you need a well-constructed, well-designed and mature data management program.
Before proceeding, let’s step back and look at the full landscape of the AI/ML program. I like to define the “legs of the AI/ML stool” as follows:
- Leg 1: Data Management
- Leg 2: Model Management
- Leg 3: (and often forgotten) Outcome Management
Let’s explore each…
Leg 1 for an AI/ML Program: Data Management
Data Management is about the supply chain of the data asset. It’s about getting the right data to the right people at the right time. It’s about understanding and having full transparency of the data’s source, understanding how it is processed and curated, where it is persisted and how it is maintained. Data that feeds our analytics, AI/ML models, must be accurate, timely and trusted. The overly used expression, “garbage in, garbage out,” sums it up best.
Curious as to where this expression of “garbage in, garbage out” came from, I did a little research and discovered that this phrase is attributed to a man named George Fuechsel, an IBM programmer in the 1960’s who emphasized that bad data in, gets bad results out. Seventy years later, this expression holds true. As smart as our AI/ML can be, it still depends upon accurate, timely and trusted data. No matter how good your AI/ML models are, they are still intrinsically connected and dependent upon data that feeds them. You can’t have good analytics, good AI/ML, without good data. A 2-legged stool will not stand!
So you see, data governance alone is not enough. To successfully manage your AI/ML agenda, you need the full complement of your data management capabilities, successfully implemented, to have a successful AI/ML program. The EDM Council’s DCAM (Data Management Capability Assessment Model) aptly describes the key elements of your data management program. It begins with a fully defined and endorsed data management strategy. How will your program be managed and sustained and what data do you need to support your business objectives? The next consideration is the program itself. Do you have the right organizational structure, with the right levels of executive backing to support your program? And do you have the right skill sets in your organization to accomplish your data management objectives? Next you need to understand the business itself. Business architecture articulates the business objectives. Business objectives define data requirements. And data requirements define technology. Once established, the important tasks of data quality and data governance can be deployed to ensure trust in your data assets. And finally, every data program must understand the nature of the data itself, safeguarding the sensitivity of personal data and protecting against unauthorized access and data breaches.
Unfortunately, I see many organizations anxious to jump into AI/ML without having an established data management program. You can lay the foundation of a building with inferior concrete, but it won’t stand the test of time. Data is the foundation of your AI/ML program.
Leg 2 for an AI/ML Program: Model Management
Next comes model management. I do not profess to be an expert in building analytic, AI/ML models, but I can assure you that the same principles that apply to data management carry over into model management. Model development must adhere to a set of well-defined principles and standards and must be carefully developed and tested to ensure they are performing as intended. While developing these models to achieve a particular business objective, the modelers must constantly be aware of detecting bias in the algorithms and ensuring fairness in their design. In November 2018, The Monetary Authority of Singapore (MAS) published the “FEAT” Principles — Fairness, Ethics, Accountability and Transparency — a guide to the responsible and ethical use of AI. This document codifies, into 14 principles, the areas of concern for every developer of AI/ML models. AI/ML is still a relatively new skill. My advice: take advantage of the subject matter experts who labored over this document, and others like it, and leverage their insights in developing the models that your firm can use to help accelerate your business forward.
Leg 3 for an AI/ML Program: Outcome Management
The final leg of the stool is outcome management. For many firms, developing AI/ML solutions ended with the model going into production. And in the past, as with traditional technology applications, after unit testing, that was sufficient. But in today’s AI/ML world, what comes out of these models must be constantly reviewed and evaluated. The difference is the ML — “machine learning.” Even the best management programs overseeing large data sets can miss underlying bias in the data. This does not minimize the role of data management, but instead recognizes that the combination of unforeseen bias in the data, combined with the power of AI/ML, can bring these unintended outcomes to the forefront. One doesn’t have to look far to see a multitude of headlines that depict AI/ML models that have gone astray. When you see stories of these out-of-control models generating unethical output, I’m certain it wasn’t the intention of the developers to have these models produce these results. So the final leg of the AI/ML stool, outcome management, is critical and necessary to keep the stool upright.
Common Sense for AI/ML
In conclusion, let me change the title of this article — from The Importance of Data Governance When Implementing AI/ML to The Importance of Good Business Common Sense When Implementing AI/ML. Bring together data, model and outcome and you will be on the path to leveraging the power and potential of this new AI/ML technology.
This quarter’s column contributed by:
John Bottega, President of the EDM Council
John Bottega is a senior strategy and data management executive with more than 40 years of experience in the industry. John began working with the EDM Council as an industry contributor in 2005 and served as Chairman from 2007 to 2014. He joined the Council’s executive team as a Senior Advisor in 2014. In 2017, he took over as the Senior Executive and today holds the title of President of the EDM Council. Over his career, John has served as Chief Data Officer in both the private and public sectors, serving as CDO for Citi, Bank of America, and the Federal Reserve Bank of New York. He also served as Head of Data Management for the Office of Financial Research at the US Department of the Treasury.