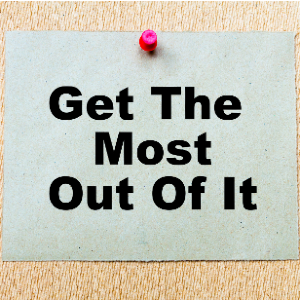
With the influx of data being generated and collected, coupled with the huge advancements in technology, it’s no surprise that organizations are leaning into data-driven approaches to stay ahead of the competition. But it’s not enough for companies to be reactive, they must be predictive.
As an example, look at how real estate industry leaders have begun to leverage data to separate themselves from the pack. Altus Group wanted to automate the appending of new data from multiple sources to a master property record.
To manage data and data access, they leveraged ThinkData Works’ Namara product to turn hundreds of disparate datasets into information assets that allow Altus to focus on product and service development. In simple business terms, they were able to automate a large proportion of their manual work in order to optimize their efficiency and productivity.
Every Industry Needs Data
Real estate isn’t the only industry that stands to benefit from transforming their data practices:
Increase profits and productivity: By neutralizing the prep and process associated with using new sources of data, insurance companies have been able to breathe new life into old models, leading to increased success in outreach campaigns.
Better decision-making: Using custom-built census profiles, pharmaceutical companies can better understand where to launch clinical trials, leading to faster product review and approval.
Increased understanding of your customers: Banks can get deeper insight on customer lifetime value and can tailor banking experience to events and customer needs.
The first step in adopting a data-first strategy is to adopt a data-first culture that ensures that the right processes and strategies are put into place. To implement this cultural shift, you must understand the basic terminology.
Data Science and Data Analytics
Data Science, in the simplest terms, involves developing methods of recording, storing, and manipulating data to extract useful information. This could be used to get retrospective insight, to predict future trends, or to find new problems.
Data Analytics is the discipline that focuses on processing existing data and performing analysis to solve problems.
These roles are not the same and are not interchangeable, so it’s important to know the difference. The data scientist is like the footage for a movie–meaningful, powerful, and important. The data analyst acts like the dialogue, bringing context to what’s on screen. Without one or the other, the project wouldn’t be complete, and it could be detrimental if lines between the two roles are blurred.
Having all the right employees and a big data lake to draw from isn’t a guarantee for success. That’s not what makes an organization data-driven. Here’s and overview of how data turns into wisdom:
Common Ground
Now that we understand what’s different between these roles, let’s look at what they share: a common pain point in data variety. Every new dataset added to a solution introduces new variables, hinders efficiency, and ultimately hurts an organization’s profitability. With so many types of data coming from a variety of sources, data professionals aren’t able to simply plug this data into their analysis. There’s a big difference between available data and accessible data. And it boils down to usability.
The Pareto Principle states that about 80% of the outcome comes from 20% of the work. By extension, 80% of your efforts lead to a mere 20% of your results. The world of data is no exception–80% of a data scientist’s time goes to prep and processing. However, clean data on its own doesn’t generate results. Just a fifth of their time is open for modelling, building, analysis, and all of the things we think of as the core of data science.
More Productive with Less Overhead
Staying the course and doing things the way they’ve always been done could be costing you a fortune. So how can businesses be the most productive with the least effort? In the case of data professionals, teams need to leverage data management tools that will save them time, save the company money, and lead to more successful project outcomes (and there are a few more reasons you need a better data management system).
You can’t just put people in a room and expect them to dance–you need some music. Your data professionals are ready, but your data has its back against the wall. A measured and planned data strategy will get the party going and allow data teams to flourish instead of flounder.
Understanding the objectives and obstacles in implementing your digital transformation efforts are key. However, to stay ahead of the competition, it’s simply not enough to meet the minimum requirements. Organizations need to develop a methodology that enhances input so they can optimize output–even when it comes to data. Getting ahead means adopting tools that increase data science productivity and efficiency so that teams can achieve their digital transformation goals faster and with more success.