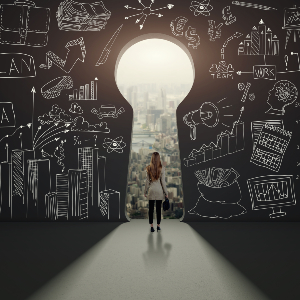
Tenets to “Thriving” As a CDAO
In the first part of this blog, I wrote about challenges Chief Data Analytics Officers face in their role. Our understanding of the challenges as background on CDAOs brings us to the four key tenets to thriving as a CDAO. They form a guiding framework to build sustainable data-driven organizations. Think about the four tenets as the blueprint or an architecture map for engineering a solid data infrastructure to lead with data when achieving business goals.
Tenet 1: Create an Effective Operating Model Strategy
The operating model is the base for any data program. It provides a perspective on how an organization is structured and the visibility of various actors in leadership roles across business lines. It is a critical input for CDAOs to consider when defining enterprise roles and responsibilities or when building a data organization. Most successful CDAOs, within the first 30-60-90 days of joining an organization, focus on defining the governing structure, aka operating model, which involves leaders across the business and information technology groups.
Depending on the organization type and its culture, an operating model takes different shapes, as represented in Figure 3 below:
In addition, defining an effective operating model is critical for any organization’s success. The operating model outlines how an organization delivers value to its customers or stakeholders, organizes its resources, and manages its processes to achieve its objectives.
An effective operating model should be aligned with an organization’s overall strategy and designed to optimize its resources and capabilities. It should also enable the organization to adapt to changing market conditions and customer needs.
The operating model typically comprises of four key elements:
- Governance: This refers to the organizational structure, decision-making processes, and policies that govern the organization’s operations. A practical governance framework helps ensure the organization’s resources are used efficiently and effectively.
- Processes: This refers to the set of activities that an organization undertakes to deliver value to its customers. Effective processes should be designed to optimize efficiency, minimize risk, and deliver high-quality data products and services.
- People: This refers to the organization’s human resources (employees, contractors, and partners). An effective operating model should provide the right talent and capabilities needed to support the organization’s objectives.
- Technology: This refers to the tools and systems the organization uses to support its operations. An effective operating model should leverage technology to optimize efficiency, enhance the customer experience, and drive innovation.
Case Study 1: Operational Excellence And Operating Model Example From A Leading Insurance Provider
Location: New York City
The insurance industry deals with sensitive customer data. However, the challenge in insurance is managing vast amounts of data from different sources and formats, including policy, claims, and customer information. The ability to extract insights from this data can help insurance companies identify risks, fraud, and other trends that can help them make better business decisions.
A CDAO at one of the major insurance providers in New York City started the data governance journey by defining the operating model. As part of the initial phase to understand various business needs, the CDAO interviewed leaders across each business line covering:
- Underwriting: This function involves assessing risk and determining the terms and pricing for insurance policies. The underwriting team evaluates the risk associated with insuring a particular person, property, or business and decides whether to provide coverage and, if so, at what cost.
- Claims: The claims function involves processing and paying out claims filed by policyholders. The claims team investigates claims to verify that the policyholder’s losses are covered by the policy and determines the payout amount.
- Actuarial: The actuarial function uses statistical analysis to evaluate risks and predict future events that may affect the insurance company’s bottom line. The actuarial team helps the underwriting team set accurate pricing for insurance policies and helps the company forecast future financial performance.
- Marketing and Sales: The marketing and sales function promotes the insurance company’s products and services to potential customers. This may include advertising, direct mail campaigns, other marketing efforts to generate leads, and sales representatives who meet with potential customers to sell insurance policies.
- Operations: The operations function involves managing the day-to-day operations of the insurance company. This may include customer service, policy administration, and information technology.
- Finance and Accounting: The finance and accounting function is responsible for managing the financial operations of the insurance company. This includes functions such as financial reporting, budgeting, and investment management.
- Legal and Compliance: The legal and compliance function ensures that the insurance company complies with all relevant laws and regulations. This may include monitoring regulation changes, maintaining compliance policies and procedures, and handling legal disputes.
For each business line, the CDAO formalized two roles to assign ownership for the business and technology areas as follows:
- Business Steward: The owners of data within each business line.
- Technical Steward: The owners of the infrastructure supporting data.
Across various business lines, stewards formed a hierarchy rolling up to the head of business lines, and business line leaders rolling up to the heads of business and IT. After several weeks of assessing the existing data culture and alignment with various stakeholders, the insurance client was ready to launch its first-ever formalized data governance structure, as shown in Figure 4 below (simplified for this article):
Note: Establishing ownership and authority are crucial steps in implementing an effective data governance program. Doing so helps socialize the program and create a cohesive structure for tackling data initiatives as a unified team.
Tenet 2: Organize Data for Better Insights by Identifying Data Domains.
Once your operating model is in place, the next critical step is to identify the data domains for each business line. Examples of common domains include customer, vendor, and product data. These domains vary across industries but ultimately serve the same purpose: capturing important information about a business and its customers. Each domain contains a vast amount of artifacts, as shown in Figure 5. Identifying data domains typically involves addressing a specific business need or issue.
Case Study 2: How One Major Financial Services Firm Tackled Data Domain Identification
Location: London
One major financial services firm dealt with vast amounts of sensitive data, including customer financial information and transactions. Therefore, data privacy, security, and regulatory compliance were significant challenges for the company. They needed to ensure that their data storage, processing, and access were secure and compliant with regulations.
As part of the exercise to identify data domains, the financial services firm started by outlining key business requirements as follows:
- Provide better customer experience through improved control and management of customer requirements.
- Streamline management of customer usage and needs for better performance and personalization.
- Boost revenue through increased upsell on storage billing cycles.
Note: Successful data initiatives requires attention to people, processes, and technology. This can be achieved by defining a data governance structure, clarifying roles and responsibilities, and leveraging a technology platform to govern important information assets.
The business needs outlined above were directly tied to underlying business problems. The client sought to gain greater control over customer visibility and understanding but struggled due to fragmented data spread across multiple systems and applications without clear ownership.
Tackling such a problem warranted the data team to trace an end-to-end data lineage process on critical reports. To ensure the accuracy of reports, the data team implemented a simple rule for watermarking reports.
Rule: If you cannot identify the data source, your report is not certified.
The data team linked the business metadata with technical metadata representing the underlying systems and applications to create a report watermark. Successfully tracing the lineage of one report enabled them to scale the model for other reports. Figure 6 below outlines a sample report lineage example:
Tenet 3: Simplifying Data Strategy by Identifying Critical Data Elements in Data Domains
After defining the data domains, we find ourselves at the pinnacle of our data management journey. Looking down from here, we can see the vast landscape of data domains, spanning across tens, hundreds, and even thousands of systems and applications, each containing numerous key reports, critical data elements, and essential business processes.
It’s easy to feel overwhelmed by this data landscape’s sheer scale and complexity. But to effectively manage and govern our data, we need to be strategic and focused. We cannot afford to boil the ocean by tackling all data artifacts at once. Instead, we must identify what’s critical to the business and prioritize those elements for closer scrutiny.
So, how do we determine what’s critical? There are many factors to consider, such as the business impact of a particular data element, its regulatory requirements, its frequency of use, and its potential for reuse in other applications or systems. We must also consider the opinions and insights of key stakeholders, including business owners, data stewards, and subject matter experts.
By taking a targeted, business-driven approach to identifying critical data elements, we can better understand our data landscape and make more informed decisions about how to manage and govern it.
Case Study 3: How a Federal Government Agency Created a Centralized Data Governance Platform for Managing and Controlling Changes
Location: Washington, D.C.
A federal government agency in Washington, D.C., faced the challenge of achieving data standardization and consistency across its vast enterprise. Their goal was to create a centralized platform that could effectively manage and control changes to their critical data assets while also providing transparent visibility into those assets.
To achieve this, the agency created a comprehensive and dynamic ecosystem that would foster collaboration and enable lifecycle management. This included implementing a robust data governance framework that provided enhanced data security and integrity, along with comprehensive audit logs for historical and future analysis.
The resulting platform helped the agency achieve its data governance objectives and improved its overall operational efficiency and decision-making capabilities. By leveraging the power of data to gain valuable insights, the agency was able to make informed decisions that positively impacted its organization and the constituents it served.
Case Study 4: Technology Company’s Report Certification Process
Location: Silicon Valley, San Francisco Bay Area
A technology company needed to validate its financial reports and related source systems. The company began by identifying ten key reports and documenting information about the system’s origin. As the initiative was scaled, it became known as the “report certification” process and applied to all reports, requiring certification and related source system information.
The report certification process ensured that reports were only certified if the owners could prove their data lineage down to the system of origin. This approach allowed the company to gain more control over its data and ensure that financial reports were accurate and reliable.
Case Study 5: How USAA Continues to Set an Industry Standard for Data-Driven Leadership By Randy Bean
Location: Forbes
I highly recommend checking out How USAA Continues To Set An Industry Standard For Data Driven Leadership” on Forbes. The article features Ramnik Bajaj, currently serving as Chief Data and Analytics Officer at USAA.
In the article, Mr. Bajaj shares some great advice on how USAA uses data-driven insights to transform how they do business. If you’re looking to learn from a leader in data and analytics, this article is a must-read.
Also, check out Douglas Laney’s latest book Data Juice: 101 Stories of How Organizations Are Squeezing Value from Available Data Assets.
Tenet 4: Demystify Control Measurements to Understand The Art of Measuring Success
After understanding data governance structures, operating models, data domains, and identifying critical data elements, organizations must establish and maintain control to sustain their governance program. With experience delivering data governance, catalog, and privacy solutions across various industries, it is evident that initiatives like data governance are not one-time projects, but rather continuous programs that empower data-driven decision-making and create business opportunities to thrive. Implementing control measurements is essential for organizations to meet business standards and ensure the success of their data governance initiatives. These control measurements involve the following key activities:
- Define automated workflow processes and thresholds for approval, escalation, review, voting, and issue management.
- Apply these workflow processes to the governance structure, data domains, and critical data elements.
- Develop reporting on the progress of steps 1 and 2, including crowdsourcing through ratings and reviews.
- Capture feedback through automated workflow processes and maintain audit trails to capture historical changes (before/after).
- Document policies and standards and link them to business and technical metadata for risk reporting.
- Document data quality and privacy rules and create data quality and privacy dashboards and scorecards for review and remediation.
Case Study 6: A Technology Company Building a Robust Data Governance Organization for Managing Business Data Definitions
Location: California
An example of successful data governance implementation is a technology company based in California. In 2010, they started by defining ownership, roles, responsibilities, and business data definitions. They also applied workflow processes that involved data stewards in the change management process.
Through these efforts, the company established a robust data governance organization that supports an ongoing program for managing all business data definitions. This includes executing control processes such as onboarding business data definitions, obtaining approval, collaborating with data stewards, and capturing feedback.
The four key tenets of thriving as a CDAO mentioned above are just the beginning when it comes to launching an enterprise-wide data strategy and data governance program. Depending on the industry, there may be different approaches to consider. However, these steps are essential for establishing effective information governance, the foundation for improving data quality, privacy, security, and maximizing business intelligence and master data management programs. Data governance is also critical for preparing for emerging trends such as OpenAI, IoT, ESG, GDPR, CCPA, etc. By acting as a toll gate, governance ensures that data is properly defined, of good quality, private, controlled, and has proper lineage before taking any initiative.
Thank you for reading this blog on the four key tenets to thriving as a CDAO. Hope it has provided valuable insights and strategies for success in your role as a Chief Data and Analytics Officer.