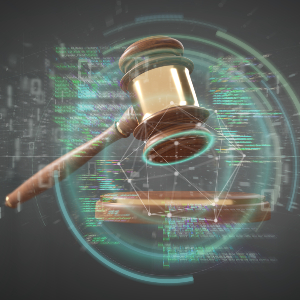
Data is the supply chain for AI. For generative AI, even in fine-tuned, company-specific large language models, the data that is input into training data comes from a host of different sources. If the data from any given source is unreliable, then the training data will be deficient and the LLM output will be untrustworthy. In this sense, the data supply chain is analogous to a manufacturing supply chain. For example, defects or impurities in raw materials or component parts will cause the finished product to fail or not perform optimally for the end consumer. The remedial efforts necessary to identify the root cause of a defect and retrofit a manufactured product are time-consuming and expensive, equally so with data-driven AI products. This article briefly outlines how the specific business goals of the company should inform the legal strategy for maximizing reliability and safe usage of data in each stage of the data supply chain.
How does one protect the data supply chain? First and foremost, by preserving data quality as defined by data professionals. Second, by ensuring that the legal protections, rights, and issues that apply to different types of data are considered at each stage of the data supply chain and are designed to ensure protected use of the data at each stage and in the final outputs. AI and data are mutually reinforcing in the sense that reliable data is essential to reliable AI outputs and AI tools can be used to improve data quality. In essence, the goal of ensuring data quality and the appropriate legal protections for that data is to ensure that you do not buy a “data museum” or invest in data that cannot be used for its intended purpose.
Adopting new technology through outsourcing or business transformations can reveal previously hidden data issues. Re-evaluation of the data supply chain, therefore, is an essential step in the outsourcing process. The risk from failing to constantly re-evaluate the strength of your data supply chain is that company personnel or third parties will lose faith in the data that drives business decisions. For example, in the oil and gas industry, unreliable data can lead to mistaken strategies for where and how often to drill an oil well. Likewise, a CFO relies on the reliable integration of sales data and metrics from other operations of the company. In either case (and many others), the data supply chain is crucial to business decisions and strategies.
There are numerous business goals and strategies that drive the acquisition and use of data:
- Data for internal use by the business (e.g., analytics, strategic planning, quality control)
- Data for commercialization
- Data sought as part of an M&A strategy
- Data as part of a divestiture, sale or license strategy
- Regulatory compliance
- Data subject to AI laws or regulations
- Due diligence generally
The particular business goal drives the framework for assessing a variety of legal issues crucial to protecting the data supply chain. First, does the company have the necessary data rights to support the business activity. Is there existing legal liability associated with the data? Will the acquiring company acquire data or a lawsuit? If there is potential liability associated with the data, can it be cured? If the data is licensed, can the license be assigned? Are there licensing restrictions that will adversely affect the company’s ability to pursue its business strategy? Are there other contractual restrictions impacting the use of the data?
Second, does the data have a history or characteristic that could impact its reliability or the ability of the Company to use it effectively? Has the data been compromised in a cyberattack? If the data is obtained from a data broker, can it be repurposed for a different type and scope of use? Is the data PII, and if so, is the scope of consent adequate?
Third, will the data be subject to new regulatory restrictions or requirements when used for the acquiring Company’s strategy? Can the acquirer use the data as part of its services in the relevant jurisdictions? Are there local regulations or frameworks that limit use of the data based on its history, new application or end user characteristics? Are there local data breach laws that impact the proposed use (e.g., autonomous driving)?
The answers to all of these questions will dictate the legal structure of data transactions and the strategies for data use, as informed by regulations and other legal considerations. While data is a business asset in a transaction, it is not a unitary asset. It is important for companies and their counsel to structure contracts by dividing the data into transaction-specific categories, with appropriate and well-thought-out rights and obligations tied to each category. These same categories can be used when the company looks to commercialize data into a new revenue stream. A category-based approach leads to licensing different data categories in different ways.
What are some of the different data categories that call for a discrete but coordinated legal and business use analysis?
- Raw Data – Includes data entered into an AI solution by the customer, data obtained from the company’s own upstream supply chain
- Stored Data – Data generated by provider and stored in its IT assets (after indexing and applying rules to raw data)
- Processed Data – Data generated by provider by running analytics on and making inferences from stored data
- Regulated Data – Data subject to regulations applicable to a transaction, such as privacy consents, “know your customer” requirements, etc.
- Regulatory Data – Data provided (or to be provided) to regulators
- Solution Data – Data provided to the customer after use of the services
- Aggregate Data – Data derived from or aggregated in de-identified form from stored data, processed data, or customer’s uses of provider services or systems
- Customer-Specific Data – Stored data or processed data that reveals data or information about a specific customer of the provider
Each of these categories of data may warrant different protections and use rights depending on the business goals the company is seeking to achieve. Accordingly, the process outlined above — identifying business goals, legal requirements and best legal practices, data-specific legal risks, and the categories of data employed — is essential for all companies with existing data supply chains and those seeking to build out a new data supply chain.
William A. Tanenbaum
William A. Tanenbaum is a data, technology, privacy, and IP lawyer, and a partner in the 100-year-old New York law firm Moses Singer. Who’s Who Legal says Bill is a “go-to expert” on “the management of and protection of data across a variety of sectors.” It named him “one of the leading names” in AI and data, and ranked him as one of the international “Thought Leaders in Data.” Chambers, America’s Leading Lawyers for Business, says Bill has “notable expertise in cybersecurity, data law, and IP,” has a “solid national reputation,” and “brings extremely high integrity, a deep intellect, fearlessness, and a practical, real-world mindset to every problem.” Bill is a member of the DAMA Speakers Bureau and the Past President of the International Technology Law Association. He is a graduate of Brown University (Phi Beta Kappa), Cornell Law School, and the Bob Bondurant School of High-Performance Driving. Follow William on LinkedIn.
Isaac Greaney
Isaac Greaney is a partner in the Litigation practice group at Moses Singer. Isaac focuses his practice on commercial litigation and disputes, with a concentration in securities litigation, and the defense of securities enforcement investigations. He helps clients navigate through complex and high-profile litigation and regulatory matters. He received his B.A. from the University of Chicago and his J.D. from Fordham University School of Law. Isaac clerked for Thomas J. Meskill, U.S. Court of Appeals, 2nd Circuit.
Isaac’s LinkedIn profile can be found here and his website profile can be found here.