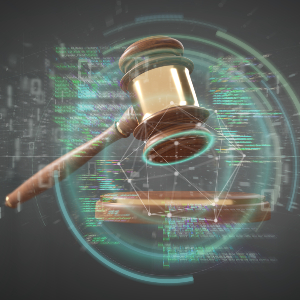
The use of Artificial Intelligence (AI) in healthcare provides promises, risks, and unintended consequences. This column addresses the evolving AI issues in connection with the following topics: As used in this column, “AI” covers both generative and non-generative AI, with a focus on machine learning as part of non-generative AI.
Reducing Administrative Burdens on Physicians
One of the most promising benefits of AI in healthcare is that it can meaningfully reduce the time physicians spend on administrative functions. This includes their time trying to reconcile data coming from different systems or generated by systems that are in a form that itself does not provide sufficient information about the subject matter of a specific patient visit. In this sense, AI can be said to be a mediator between existing IT systems with their design-in limitations and the data that physicians need to extract from the output or on screen presentation of the data.
Reducing administrative burden will free up time doctors now are required to spend not interacting with their patients. Anecdotally, this will increase doctor satisfaction and allow physicians more time for direct interaction with patients during appointments. For the same reason, using AI and concomitant data governance will increase patient satisfaction for the same reason. Even in the simple situation of using AI to coordinate appointments will lead to benefits in how medical care is explained and delivered. Put simply, healthcare AI can increase not only the quantity of direct physician-patient time, but also the quality of that time, or, put another way, let doctors be doctors and not virtual IT staff.
Reducing the administrative burden at the individual physician level requires using AI at the equivalent of the enterprise or business unit level. This, in turn, requires not only good data practices and relevant AI practices, but the legal agreements that implement practices through proper documentation into a technology and data-rich ecosystem with multiple stakeholders with differing expectations. The legal agreements, together with the inter-enterprise policies that are the equivalent of agreements, are used to allocate responsibilities and accountability. Turning the focus from the physician to a focus on a hospital or a medical practice as a whole, the promise of using AI to reduce administrative overhead at the enterprise level with the promise of AI is that it will reduce the administrative burdens at the enterprise level as well.
Best Medical Practices Part I: Faster Dissemination
AI is used in the development of new best medical practices, including incremental or substantial revisions or improvements to currently followed best practices. Best practices, by their nature, improve with advances in research and clinical studies. Not only can AI speed the development of new best practices, but, as is important for the scope of this article, AI has the capacity to speed the dissemination of newly developed best practices to physicians and medical centers over traditional publication of journals and medical papers. Speeding dissemination can also lead to speeding verification and adoption of best practices.
Best Practices Part II: Freezing Best Practices in Place
This is also a potential adverse effect of AI on the use of medical best practices. AI can be used to “codify” best practices in establishing standard steps to be used in medical protocols in standard medical scenarios. A simple example of this may be the steps that EMTs follow as a matter of protocol when transporting patients to the hospital who are identified as having a specific medical condition. The risk created by AI is that once a best practice is embedded in AI used to promulgate protocols, it becomes a best practice that may not be subject to timely revelation and removed from and replaced by another better practice as part of a standard protocol. In other words, AI-generated protocols may have the unintended effect of taking a best practice and freezing it in place as the current best practice when the practice has been superseded. Thus, while AI can create and disseminate new best practices, as indicated in the preceding section of this article, the healthcare profession will be cognizant of the steps needed to “import” new best practices into standard protocols.
AI and Risk of Systematic Failure
In a scenario where a single MRI or a similar medical device fails, the impact can be characterized as a one-device, one-patient-at-a-time failure. If devices are connected and/or depend on a common AI solution, and the solution fails, then the result is not a one-patient-at-a-time failure, but a systematic failure affecting a large number of machines and patients in near real time. This risk can be accelerated as the Healthcare Internet of Things creates an integrated system used to deliver medical care.
A systematic failure can have two adverse consequences. First, it is difficult to reschedule patients who had appointments for treatment dependent upon systems for sophisticated treatment or who need specialized care provided by an AI-enabled system. This delays treatment and complicates rescheduling of treatment for seriously ill patients. In addition, the hospital loses income when the system is not being used. The hospital cannot get reimbursed for procedures it does not perform.
Because the failure is systematic, more of a remedy is required than having technicians who can remove a machine from service while it is diagnosed and repaired. Where AI can create a systematic failure, another solution is required. From both operational and legal perspectives, this means that healthcare systems should have contractual obligations to have a SWAT team available to analyze and restore the system.
Conclusion
Technology and data-based evidence is always used to practice medicine. AI is a new layer of technology that has been proven to speed research and improve clinical care. It provides benefits, limitations, and potentially intended adverse consequences.