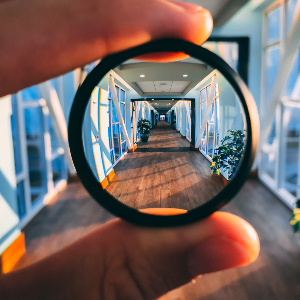
“What’s a metaphor?”
Mr. Biergel posed the question one morning to my high school grammar class. Being typical teenagers, we looked at him with blank-eyed stares. We expected that if we waited long enough, he’d write a paragraph-long definition on the blackboard.
“What’s a metaphor?” he repeated.
“A place for cows to graze!”
We groaned. Audibly. Another one of Mr. Biergel’s terrible puns.
Far too many years lie between today and that class. I remember that joke despite having forgotten much of my high school education. I do think Mr. Biergel was an excellent grammar teacher. I am sure I developed my abhorrence of passive sentences from his class.
So, where is this going, data-wise? Well, just keep the thought of cows grazing in mind — we will return to that.
A metaphor, per the Oxford English Dictionary, is certainly not a meadow:
“A figure of speech in which a name or descriptive word or phrase is transferred to an object or action different from, but analogous to, that to which it is literally applicable.”1
Now that’s clear as mud — which in itself is a metaphor. A few more examples:
- All the world’s a stage
- Couch potato.
- A heart of gold.
You get the sense of how pervasive metaphors are in everyday communication. James Geary in his TED talk said we use six metaphors a minute in conversation.2 Xiaoyu Tong, Rochelle Choenni, Martha Lewis and Ekaterina Shutova write in their paper, “Metaphor Understanding Challenge Dataset for LLMs,” that “LLMs [Large Language Models, such as ChatGPT], therefore, require the ability to comprehend metaphor in order to have a full command of language. As such, metaphor understanding is an essential task for evaluating the capabilities of LLMs.”
I’ll dive into this paper in detail later. For now, let’s revisit Umberto Eco, who has figured prominently in my last two columns. Eco titles his most interesting chapter in “Semiotics and the Philosophy of Language,” “Metaphor.” Eco begins by explaining how hard it is, despite “the thousands and thousands of pages written about the metaphor,”3 to define what a metaphor is. “The chronicle of the discussion on metaphors is the chronicle of a series of variations on a few tautologies, perhaps on a single one: “A metaphor is an artifice which permits one to speak metaphorically’.”4
Eco lays out a history of philosophical thought on metaphors, beginning with Aristotle. Yes, that Aristotle, the one from Ancient Greece, circa 350 B.C. The author of “Poetics” and “Rhetoric,” and teacher of Alexander the Great. As a semiotician, Eco is interested in a metaphor as a linguistic sign and how the listener or reader interprets it. Eco notes that “What Aristotle understood was that the metaphor is not an ornament (κόσμος), but rather a cognitive instrument, at once a source of clarity and enigma.”5
Aristotle himself puts it this way: “Now strange words simply puzzle us; ordinary words convey only what we know already; it is from metaphor that we can best get hold of something fresh…. When the poet calls ‘old age a withered stalk,’ he conveys a new idea, a new fact, to us by means of the general notion of bloom, which is common to both things.”6
Eco, writing in 1984, concludes the chapter with what today might sound like a bold claim: “No algorithm exists for the metaphor, nor can a metaphor be produced by means of a computer’s precise instructions, not matter what the volume of organized information to be fed in.”7
Not so, John Nosta would argue. In his article on LLMs in…wait for it…“Psychology Today.” In “Echoes of Aristotle: LLM’s Mastery of the Metaphor,” Nosta states as key points:
- LLMs meld diverse knowledge, arguably embodying Aristotle’s metaphorical genius.
- “Techno-connectivity” in LLMs parallels human intellectual traditions, crafting insights from vast knowledge.
- LLMs’ pattern recognition mirrors human cognition, extending Aristotle’s genius concept to digital innovation.
- LLMs democratize genius, transforming creative insights across fields by connecting unrelated information.
“Democratize genius?” I thought democratizing data was ambitious.
Nosta includes a quote from Aristotle’s “Poetics” (though Nosta doesn’t bother to provide a citation — I had to find the source myself). In the translation of “Poetics” I own, the quote reads: “But the greatest thing by far is to have a command of metaphor. This alone cannot be imparted by another; it is the mark of genius, for to make good metaphors implies an eye for resemblances.8
If LLMs have “mastery of the metaphor,” how effective is their interpretation? I found several papers on this topic, two of which I’ll summarize here. I’m not a researcher and can’t comment on the quality of the survey and analysis techniques these papers’ authors employ. Both papers provide links to their datasets, LLM prompts, and code. I will share my impressions of the relative complexity of the test data, and my perception of the authors’ objectivity or lack of same.
First, “Large Language Model Displays Emergent Ability to Interpret Novel Literary Metaphors” by Nicholas Ichien, Dušan Stamenković, and Keith J. Holyoak. This paper evaluates the metaphor interpretation capabilities of ChatGPT-4. The authors discourse on their approach, which includes building a dataset of “novel” metaphors. to reduce the possibility ChatGPT is familiar with them9 These include Serbian poems translated into English as well as “entire novel English poems.” The authors explain the “science” behind literary metaphors:
Computational analyses have shown that literary metaphors are distinguished by the qualities of high surprisal (a statistical measure of the unexpectedness of words), relative dissimilarity of source and target concepts, the combination of concrete words with relatively complex grammar and high lexical diversity, and extra difficulty (for people) in comprehending the metaphorical meaning (Baggio, 2018; Jacobs & Kinder, 2017, 2018).
I recalled as I read this my favorite moment from the movie Dead Poets Society. The late Robin Williams, unforgettable as Keating, the teacher. Keating asks a student to read the Preface of a volume of verse, “Understanding Poetry” by Dr. J. Evans Pritchard, Ph.D. Pritchard describes how to determine a poem’s value by graphing two factors on a graph.
Keating interrupts the student: “Excrement. That’s what I think of Mr. J. Evans Pritchard. We’re not laying pipe. We’re talking about poetry. How can you describe poetry like American Bandstand? ‘Oh, I like Byron. I give him a 42, but I can’t dance to it.’”
Keating goes on to have the boys rip the introduction out of the volume. As I have a PDF of this paper, I had nothing to tear. Sadly.
But I digress. Next the paper describes the process of assessing the LLMs’ understanding of metaphors. First, the team measures interpreting the dataset of metaphors from Serbian poets. The authors state that GPT-4 had comparable results to human students. The metaphors in the dataset are simplistic, based on a brief review. For example, “A waterfall is a wild, unbridled horse,” and “Love is radiance.” Given this, I did not find the results so impressive.
More telling: the results of interpreting novel (new) English poems. One of the human poets reviewed GPT-4’s interpretations of these poems. This person “was blind to the origin of the interpretations and was not told that an AI system was involved.”
Although the “human critic” did say GPT-4’s interpretations “accurately expressed the symbolic meaning of the poem, [he] expressed important reservations related to emotional sensitivity. After finishing all eight assessments, [he] spontaneously provided an overall characterization of the ‘critic’ being evaluated (i.e., GPT-4):
The critic [GPT-4] in all these interpretations zones in on the themes and meanings and their interconnections, but he is weak on evocation portraying just exactly how the reader feels the poem…. This suggests to me that the ‘critic’ relies on a formula and perhaps is an AI program. It just seems to be without any flair at all even though as far as the straightforward features of a poem goes, it is all correct. It is like a meal that looks done right, even beautifully appealing, but without zesty taste.
For reasons not stated, the authors decided to have the human critic evaluate poems written by GPT-4. “His evaluations of GPT-4’s literary efforts can be characterized as scathing. For example, the human critic describes GPT-4’s variant of one poem as ‘happy rhyming akin to Sunday school joy.’ Lack of emotional depth, a perceived weakness of GPT-4’s otherwise excellent interpretations of human poems, may pose a serious impediment to its capacity to generate novel poems with any literary merit.”
You think?
Moving on.
“Metaphor Understanding Challenge Dataset for LLMs,” by Tong, Choenni, Lewis, and Shutova. I quoted this paper earlier. It is more objective in tone than the previous paper. It also offers up a dataset of impressive scope and breadth:
The dataset provides over 10k paraphrases for sentences containing metaphor use, as well as 1.5k instances containing inapt paraphrases. The inapt paraphrases were carefully selected to serve as control to determine whether the model indeed performs full metaphor interpretation or rather resorts to lexical similarity. All apt and inapt paraphrases were manually annotated. The metaphorical sentences cover natural metaphor uses across 4 genres (academic, news, fiction, and conversation), and they exhibit different levels of novelty.
This is the Metaphor Understanding Challenge Dataset, or MUNCH. Which is something I am sure cows do as they graze. See how it all comes together?
As mentioned, both papers’ methodologies attempt to offset LLMs’ familiarity with metaphors included in their training data. Ichien, Stamenković, and Holyoak address this by using Serbian metaphors translated to English as well as new English poems. Tong, Choenni, Lewis, and Shutova use a “novelty criterion” and scored each metaphor, excluding those with the lowest scores from the dataset. The researchers deployed crowdsourcing to identify apt paraphrases of each metaphor in the dataset. They used a combination of Wordnet10 and input from a PhD candidate in linguistics specializing in metaphor research to develop “inapt” paraphrases for each metaphor.
The paper evaluates LLaMA-13B, LLaMA-30B, and GPT-3.5. I note that comparing the GPT results here with those of the first paper which studied GPT 4.0 must consider the differences between the releases. I look forward to seeing the just-released ChatGPT 4o attempt to graze on MUNCH.
Tong, Choenni, Lewis, and Shutova demonstrate a deep linguistic understanding of metaphors. For example, they write in the introduction:
Metaphors are linguistic expressions based on conceptual mappings between a target and a source domain (Lakoff and Johnson, 1980). The verb phrase to stir excitement, for example, is based on the conceptual metaphor FEELING IS LIQUID, with FEELING excitement) being the target domain and LIQUID (something that can be stirred) the source domain. The metaphor compares FEELING with LIQUID, introducing vividness into the description of an otherwise intangible emotional impact. Such cross-domain mappings are sets of systematic ontological correspondences, mapping concepts and their relational structure across distinct domains.
The authors evaluate the LLMs on two tasks:
- Paraphrase judgement, requiring the model to select correct paraphrases for a given sentence from given candidates.
- Paraphrase generation, asking the model to generate correct paraphrases for a given reference sentence.
The paper details the prompts used for both tasks. The researchers note that the results for (1) were below the random baseline in most cases and for (2), ChatGPT performed best at generating paraphrases which matched human answers, but “all three models clearly preferred different answers as compared to human annotators.”
The authors conclude their analysis with a thoughtful statement:
To sum up, the results of the two paraphrase tasks indicate that the LLMs are unable to (fully) understand some of the metaphors in our dataset. The paraphrase judgement task further reveals that the models have difficulty distinguishing the metaphors’ source domains (implied by the inapt paraphrases) and target domains (implied by the reference sentences and apt paraphrases)…This means that for downstream NLP tasks such as opinion mining, bias detection, humour detection, and intent recognition, the LLMs could overlook the entailment of a metaphor. In machine translation as well as summarisation of highly figurative or poetic texts, the problems may manifest as incorrect or peculiar explanation of metaphors.
This hints at the depth Eco writes about metaphors as “cognitive instruments.” It also echoes knowledge graphs and ontologies. In Part II, I’ll follow where this leads. How does a knowledge graph’s vocabulary of “triples” relate to metaphoric thinking? Will I challenge ChatGPT 4.0 myself to see if it displays an understanding of metaphors to the degree Eco depicts in his interpretive rules? Stay tuned!
1 “Metaphor, N., Sense 1.” Oxford English Dictionary, Oxford UP, March 2024, https://doi.org/10.1093/OED/2147136838.
2 ted.com/talks/lang/eng/james_geary_metaphorically_speaking.html
3 Eco, Umberto, Semiotics and the Philosophy of Language, 1984, First Midland Book edition 1986, pg. 88.
4 Eco, Ibid, pg. 88.
5 Eco, Ibid. pp. 91-95
6 Aristotle. Rhetoric (Start Publishing) (pp. 183-184). Start Publishing LLC. Kindle Edition.
7 Eco, Ibid. pg. 127
8 Aristotle. Poetics (Dover Thrift Editions: Philosophy) (p. 37). Dover Publications. Kindle Edition.
9 Since OpenAI does not disclose its LLMs’ training data, researchers cannot determine whether or not the training dataset included these metaphors.