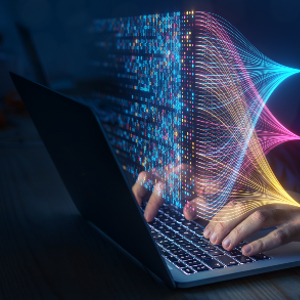
In the rapidly evolving data landscape, data practitioners face a plethora of concepts and architectures. Data mesh argues for a decentralized approach to data and for data to be delivered as curated, reusable data products under the ownership of business domains. Meanwhile, according to the authors of “Rewired,” data fabric offers “the promise of greatly accelerated and cheaper integration through virtualization to connect data sources to the data fabric without necessary data movement.”
Among these, the modern data stack (MDS) stands out as a beacon, guiding organizations through the complexities of data management and utilization. It’s not just another buzzword; it’s an essential reference architecture for anyone serious about leveraging data as a strategic asset. In this article, I’ll share the origins for modern data stack thinking, what a modern data stack provides, observations on what it misses, what CIOs hope to gain, the importance of adding GenAI, and what is needed to update the architecture for today’s data era.
The Genesis of Modern Data Stack
The term “modern data stack” was coined as cloud data warehouses (CDWs) rose to prominence. Pioneered by the founders of FiveTran, it signified a shift from the legacy extract, transform, load (ETL) processes to an Extract, Load, Transform (ELT). But at its core, it reflected the decoupling of storage and processing services. This paradigm necessitated a redesign of data operations, demanding fresh data management strategies, and a high degree of ecosystem interoperability. An influential work by Andreessen Horowitz on “Emerging Architectures for Modern Data Infrastructure” sought to bridge the gaps with a complete reference architecture.
Pillars of a Modern Data Stack
The MDS orchestrates the flow and management of data across platforms, recognizing the need for distinct services in an ever-expanding data environment. It’s not just about managing data; it’s about facilitating discovery, governance, observability, and ensuring data security and entitlements are applied across the board. But it’s not without its limitations.
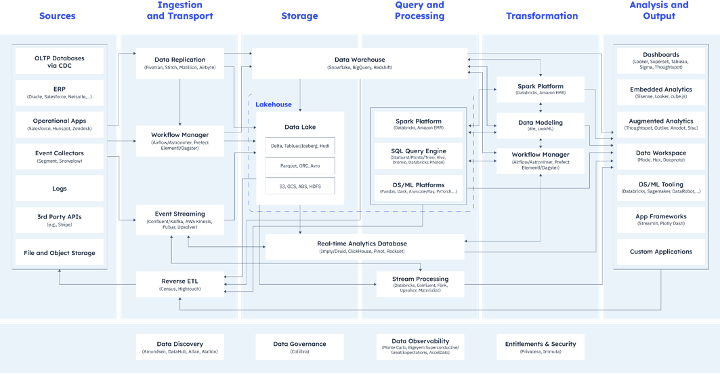
Observations on the Limitations
Despite its comprehensive nature, the MDS has areas that require further development:
- Data discovery: Data discovery is about a lot more than data catalogs. It needs to include the discover of data, the discovery of that data’s lineage, and the discovery of sensitive data.
- Data governance isn’t a standalone function: Data governance is needed for data discovery and glossaries, data observability, data lineage, and entitlements and security.
- Policy and control integration: Policies must seamlessly link to controls to manage and measure data effectively.
- Manage and measure: Manage and Measure is a core element of DataOps/Continual Improvement Thinking. As a goal, organizations should measure period over period for improvement. Metrics include items such as data stewards established, data estate discovered, data policies defined, and data access controls established.
- Generative AI (GenAI): MDS needs to fully embrace, GenAI and its unique challenges in model management and security.
The CIO and CDO Vision for the Modern Data Stack
CIOs and CDOs seek to elevate data to a strategic level, enhancing accessibility and driving business outcomes through digital transformation. They aim to dispel the mess of technical debt, shatter data silos, and foster a robust data culture. Dion Hinchcliffe, a VP and principal analyst at Constellation Research, encapsulates this vision, advocating for a systematic approach to managing a data fabric across all clouds. He says, “Data leaders need a way to systematically create and manage a data fabric across all clouds, with local variation only occurring when required.”
Adapting MDS for the Generative AI Era
To stay relevant, the MDS must evolve. This involves:
- Refining persistent services: Clarifying the role and operation of persistent services within the stack.
- Incorporating measurement and monitoring: Adding layers to continuously monitor and measure data handling performance.
- Expanding function descriptions: Detailing the necessary actions at each function level to accommodate GenAI considerations.
- Integrating LLM and AI tools: Including language models, prompts, and chatbots as integral components of the data stack.
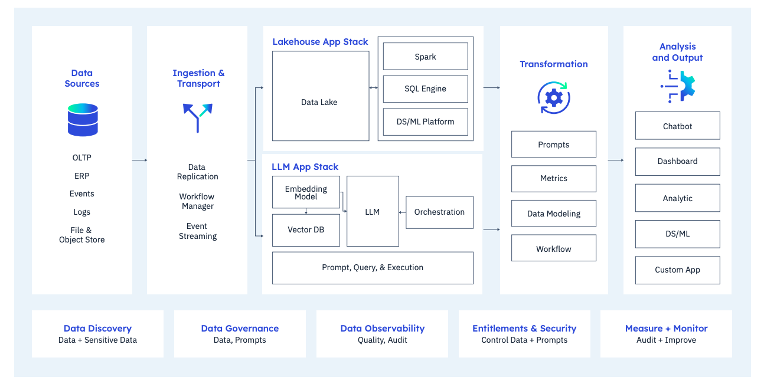
Conclusion: The Holistic Approach
The MDS is not just a guide; it’s a framework that empowers organizations to position data at the forefront of their strategic operations. It outlines the layers of the much-discussed data fabric and by incorporating GenAI, it becomes a comprehensive solution, ready to take on today’s data challenges. As data practitioners, we must embrace and continually adapt the MDS, ensuring it reflects the dynamic nature of our digital world. Only then can we unlock the full potential of our data assets and drive innovation this includes with generative AI.