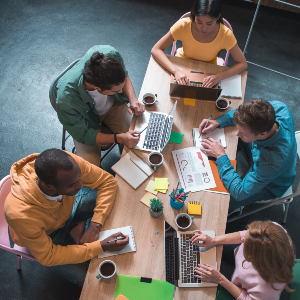
By now, it is clear to everyone that AI, especially generative AI, is the only topic you’re allowed to write about. It seems to have impacted every area of information technology, so, I will try my best to do my part. However, when it comes to data curation and data quality management, there seems to be somewhat of a conundrum.
On the one hand, there are many people claiming that having quality data is essential for having successful AI models. For example, an article in TechTarget titled “How Data Quality Shapes Machine Learning and AI Outcomes” contends that “data quality directly influences the success of machine learning models and AI initiatives.”
While most of the conversations around AI talk about how it can make almost all types of information processes work better, it seems that data quality stands out as one of the keys to making AI work better. A recent article in Information Week titled “Can Generative AI and Data Quality Coexist?” likens the need for quality data as input for AI to the same benefit that humans get from eating healthy foods, i.e., data as food for AI. A similar article in Forbes cites data quality as the real bottle neck in AI adoption calling out problems with data preparation, data debugging in model training, and data quality monitoring for deployed models.
AI models have also exposed new data quality issues that were not even on business and IT manager’s radar a few years ago like for example, model bias. Models trained on biased data can give biased results. I find it remarkable how Rich Wang and Diane Strong were so prescient in 1996 when their research identified Believability, Objectivity, and Reputation as intrinsic dimensions of data quality in addition to the more conventional dimensions such as accuracy, completeness, and consistent representation (Beyond Accuracy: What Data Quality Means to Data Consumers, Journal of Management Information Systems, Vol 12, No. 4). And conversely, do we need new data quality dimensions and metrics for AI models?
Recently, Tom Davenport and Randy Bean published the results of a survey they conducted in the Harvard Business Review. Their overall conclusion was that generative AI is making companies more data-oriented. However, at the same time, the Wavestone 2024 Data and AI Leadership and Executive Survey found that only 37% of the companies surveyed believe that their efforts to improve data quality have been successful. The same survey reports that only 63% of respondents had safeguards and guardrails in place for generative AI to govern issues such as model bias and data leakage.
On the other hand, many people are speaking out to say that AI, especially generative AI, is the answer to improving data quality. Depending on how you look at it, this is either a conundrum or the start of a great positive feedback loop. So, if improving data quality makes better AI models and better AI models improve data quality, do they ever converge? Let’s dig a little deeper.
While generative AI is very impressive, I don’t think most companies want to use it to process their payroll. As we all know, the best use of current generative AI models is not for routine calculation, but for qualitative evaluation. And it sometimes makes things up, or as they say, hallucinates. So, if you are not going to use it to profile data or other data quality tools, how is it going to improve data quality?
Well, there are some ways. A generative AI model might not do a good job of profiling a data file, but it can quickly generate and run profiling code. This could make this basic data quality process more accessible and usable by non-technical personnel, perhaps becoming a tool in the data literacy toolbox. Another way might be to ask it to evaluate data profiling results. Vendors are already using this technique to generate data quality validation rules by suggesting things like, what level of numeric deviation should be considered an outlier value for a numeric field or what could be an invalid category value or value combinations across fields. I recently saw a presentation about the ways generative AI could assist with data quality, and almost every suggestion was using it to generate code to run a standard data quality process such as profiling, outlier detection, parsing, or standardization.
While this can be very helpful, it does highlight that generative AI is mostly being used indirectly to improve data quality by improving or augmenting traditional data quality processes as opposed to directly correcting data. However, there are some exceptions to this rule that are emerging.
One of these areas relates to unstructured data. If it is true, as generally claimed, that 80% of an organization’s data are in unstructured documents, then this could be where generative AI shines as a direct contributor to data quality. Potentially AI models can be built that will perform named entity recognition (NER) more accurately and comprehensively than the current rule-based models. A NER system identifies the entities (persons, places, things) named in a document and labels them as to their specific role. For example, analyzing a free-text business news feed in which ABC Inc. is identified not only as a company, but is also annotated as the purchaser, DEF LLC is a company labeled as the company that was purchased, and the date June 16, 2020, is labeled as the date of purchase. More accurate and comprehensive NER systems could extract a treasure trove of information hidden in reports, contracts, agreement, and other types of documents.
Another way is to create realistic synthetic data for system testing and validation. When designing and testing systems that process sensitive data such as name, address, date-of-birth, social security numbers and other personally identifying information (PII), acquiring real-world data for testing and validation can be a challenge. This has always been a problem in my own area of master data management. It is also difficult to synthesize data that emulates real-life scenarios such as switching between given names and nicknames, maiden names and married names, misspellings, typing errors, OCR errors, individual and household changes of address, and name distributions that mimic a particular population. AI models able to generate data like this could provide a tremendous reduction in testing time and effort and result in the development of more robust and resilient systems.
These are just a few ways that AI could assist data quality improvement. Others include sentiment analysis, data classification, duplicate data detection, and user communications. As many professors have discovered, generative AI is really, really good at writing. It could be employed to create more readable data quality assessment reports or data quality policies and standards.
The list could go on, but I posit that the answer to the title question is that both are true. Data quality improvement is important for AI, and AI has the potential to be an important contributor to data quality improvement. They have not yet merged, but they are experiencing a positive, symbiotic relationship. And overall, that is good news for all of us as data leaders. AI is elevating the priority of data quality management and giving it new life in the organization. And as I have mentioned many times in previous columns, there is a ready-made data quality management standard ready to plug into your data governance program, the ISO 8000 Part 61, Data Quality Management: Process Reference Model. I welcome your feedback.