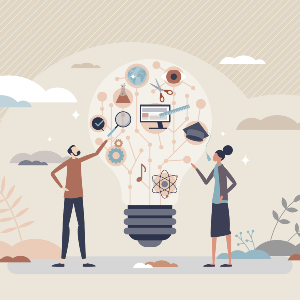
Last week, we presented a webinar in our Data Governance — Best Practices series on data quality. Among the recommended practices were identifying the specific type of data quality issue (or issues) you’re facing, prioritizing the needed changes/outcomes, and developing a process to engage users at various points in the data lifecycle. Obviously, we’re a little biased, but we believe that we offer some very useful practical suggestions that can be used by organizations, no matter where they find themselves in their data governance and data intelligence maturity. This blog post will provide some thoughts on data quality including dimensions.
One key observation from that webinar is that the suspicion or perception of poor data quality is just as powerful as the reality. We suspect that everyone agrees that having high-quality data is important. But why does data quality matter?
One answer to this question goes like this: When we cannot be certain of at least a certain level of quality in our data, it’s more difficult as an organization to use data to inform decisions, evaluate programs, propose new initiatives, or even perform daily operations. Again, though, why, exactly, is this the case? If data is considered unreliable, or incomplete, or simply not definitive, then it will be natural for people to discount it, or to use it only in certain situations, or to trust in other problem-solving methods and resources. Think about how difficult organizations find it to utilize data effectively even when they know that it’s impeccable— now think about those organizations in the face of even more indeterminacy.
In the webinar, we described a process to programmatically improve data quality, or to minimize data quality errors. It involves avenues for users to report issues, it involves the creation of data quality rules or standards, and it involves regularly profiling and assessing data to find potential problems.
We have been thinking a little about perceived data quality issues, which may or may not reflect actual problems with data capture and storage. It’s not a perfect analogy, but when users report data quality issues that don’t actually represent problems with data quality, this reminds us of a type II error with statistical hypothesis testing (“false negatives”). We have to take these reports seriously, even when our investigation reveals the quality of the data in question to be fine. But this work consumes time, often the time of highly trained (i.e., expensive) employees, and it has an opportunity cost as well.
What can organizations do to address the perception that data quality isn’t good (or good enough)? How do they reduce the incidence of these false negatives being reported? And how do they build more trust in and dependence on data?
One thing they can do is to have a process to report, investigate, and resolve data quality issues. Over time, one or both of the following is likely to happen: actual data quality problems are discovered and taken care of, so the error reporting rate will decline; or, reported data quality issues reflect not a problem with the data, but a problem with users’ knowledge of the data, and the investigations into and discussions of these reported non-issues will increase the data knowledge held by these users. By the way, it’s worth noting that organizations should encourage the reporting of perceived data quality issues, even when there is reason to believe the report is erroneous. We want everyone taking data seriously and attempting to use it as much as possible!
Another thing organizations can do is to locate some level of data quality responsibilities in their data stewards. Many organizations have widened their definition of data steward so that these people are expected not only to manage access to data they control, but to define that data publicly for the benefit of the rest of the organization. A natural next step would be for data stewards to develop, share, and, where possible, enforce data quality standards related to these data definitions. Longtime data stewards will probably have internalized basic rules about what represents quality data in their domain, but stewards new to the organization or the role, or both, may need some guidance and directed training in this work.
A third thing organizations can do is to enhance data literacy in individuals and across the organization in general. We’ve written about data literacy at some length here, but remember that it involves the ability to understand data presented in multiple forms, to interact with data meaningfully in a business context, to communicate information using data, and to ask informed questions about it. Let’s look at some generally accepted dimensions of data quality to see how higher levels of (more sophisticated) data literacy can affect perceptions of data quality.
The dimension most well understood by users is accuracy, which is a way of describing how well our data reflects (external) reality. (In our experience, the least data-literate users tend to think that data quality begins and ends with its accuracy.) Inaccurate data is likely to give rise to incorrect operating reports and misleading analytics, and so there are strong arguments in favor of doing as much as possible to maintain accurate data stores. Still, an application of superior data literacy could be instructive, as in this example. Maybe I come in to our meeting with a count of 1495 products sold, and you come into the same meaning with a count of 1505. High data literacy in a governed data environment would help us all know the following: a) whether you and I are counting the same thing; b) whether you and I followed the same rules to extract these counts; and c) whether the difference between our counts is negligible and insignificant, or whether it is significant,1 indicating that we need to look more deeply into this difference.
Completeness, another common dimension of data quality, is an interesting case as well. For some data elements, it may be a trivial exercise to tell how close or far we are to 100% completeness. If we have 10,000 address records, and each record consists of 10 fields, then we expect to have 100,000 pieces of data. However, setting a threshold here could be both arbitrary and meaningless: what if our threshold is 95%? Does that mean we have 95,000 pieces of address information? Or does it mean that for 9,500 records we have all 10 fields populated? And there are probably many pieces of interesting data where it’s difficult to assess completeness. Let’s say we want to see how much use a building or a space gets, but some of the people who have access use digital key cards while others use physical keys. As a new business analyst who doesn’t know about these legacy physical keys, I may think I’ve developed some interesting insights into using our spaces differently, or resetting some HVAC controls to save money, or who knows what. A naive understanding of data completeness would reject my new analytics out of hand, because I didn’t have all the data! But what if key card swipes represent 90% of all entrances and exits? What if there is no difference between when key card users and physical key users access buildings? Maybe my insights merit further investigation, or maybe I need to learn more about the data I’m trying to analyze.
The dimension known as timeliness is generally understood as the size of the gap between observation and recording of some data fact, or between recording that fact and its availability in data tools. But if we consider timeliness to also include how current or up-to-date our data is, or to include how long it takes to perform a thorough analysis of data, we can start to see how this could affect our ability to utilize data in the course of our work. In the example above, maybe I produced my count at 3:00 yesterday afternoon in preparation for today’s meeting, and you ran the same query at 9:30 this morning after the data warehouse refreshed, or even after some overseas sales. Of course, maybe it would be better to identify which of us is supposed to be the provider of this data, and when, so that we could avoid duplication of effort and confusion of results altogether! An organization where data literacy is high recognizes not only differences between point-in-time data snapshots, but also clarifies whose role it is to provide (summaries of) certain data in certain environments.
Having high data quality doesn’t necessarily mean that you have 100% accurate data, or 100% completeness in your records, or no data recording latency. High data quality means that you have sufficiently accurate, complete, timely, and consistent data to engage confidently in regular operations and to provide informative analytics. Getting to that point will involve identifying key data quality thresholds as an organization, defining the standards and expectations applicable to meeting those thresholds, and educating users at all proficiency levels in business data terminology, general parameters for data ranges, and appropriate computational and analytical techniques.
[1] We are not using significance in the statistical analysis sense, but rather in a more colloquial way.