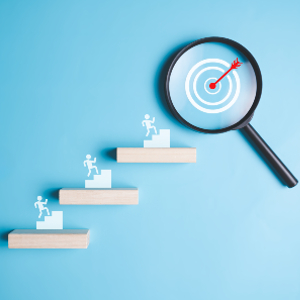
Where exactly within an organization does the primary responsibility lie for ensuring that a data pipeline project generates data of high quality, and who exactly holds that responsibility? Who is accountable for ensuring that the data is accurate? Is it the data engineers? The data scientists? The team responsible for data governance? The data analysts? The quality assurance team? Or is it all of the data teams?
Your data governance department should be responsible for ensuring data quality in a data pipeline project if all the boxes below are checked. Here are five reasons to support this choice:
- Ownership of data standards and policies: The team responsible for data governance establishes and maintains the organization’s data standards, policies, and procedures. This falls under their purview of responsibilities. They decide what it means to have “quality” data and what constitutes it, so they are the ultimate authority on how data quality should be measured and ensured. This makes them the ultimate authority on how data quality should be measured and ensured.
- Holistic view of organizational data: Data governance offers an all-encompassing perspective of an organization’s data landscape, unlike other roles, which may concentrate on particular pipeline components or datasets. This all-encompassing viewpoint ensures that data quality standards are maintained uniformly across the board, irrespective of the data’s point of origin or final destination.
- Cross-functional collaboration: Data governance acts as a bridge between various departments, ensuring that data quality standards are communicated throughout the organization, understood by all employees, and adhered to consistently. They ensure everyone is on the same page regarding data quality goals by communicating with data engineers, data scientists, analysts, and quality assurance teams.
- Testing, enforcement, and auditing: Data governance teams are responsible for the enforcement of data quality standards in addition to defining the standards themselves. They perform routine audits and assessments to ensure that data pipelines are following the standards that have been established. They also take corrective actions if discrepancies are discovered.
- Accountability for compliance and risk management: Regulations on data apply to a wide variety of industries. Teams responsible for data governance ensure that data pipelines adhere to the regulations, reducing the likelihood of facing legal repercussions. They are responsible for understanding these regulatory requirements and ensuring that data quality is maintained under those requirements.
Even though other roles, such as data engineers, data scientists, analysts, and quality assurance, play an essential part in maintaining data quality, the data governance team is ultimately responsible for the data quality in a pipeline project. This team is responsible for setting the standards, ensuring consistency, and holding the ultimate accountability for data quality.
A Process to Implement Data Quality Ownership Among Data Governance Tasks
A structured procedure is required for a data governance role or department to effectively oversee and monitor the quality of the data that is being produced as part of a data pipeline project. This procedure should incorporate strategic planning, collaboration, the utilization of technology, and ongoing reviews. The following is an in-depth procedure that exemplifies how effective data governance can be in managing data quality.
Define Data Quality Framework
Objective: Establish a clear framework for what constitutes data quality in the organization.
- Identify key data quality dimensions like accuracy, completeness, timeliness, consistency, and reliability.
- Define metrics or KPIs for each dimension to measure data quality quantitatively.
Collaborate with Stakeholders
Objective: Ensure alignment across departments regarding data quality expectations and standards.
- Engage with stakeholders who include data engineers, data scientists, business analysts, and operational teams.
- Gather information to comprehend the specific data requirements and challenges of each department.
- Educate stakeholders on the importance of data quality and the role of governance.
Implement Data Quality Tools
Objective: Encourage technologies to automate data quality checks and monitoring.
- Investigate and invest in data quality software or platforms that can be incorporated into your data pipelines.
- Use data profiling, validation, and cleansing functions to maintain high-quality data.
Set Up Continuous Monitoring
Objective: Ensure real-time or near-real-time tracking of data quality.
- Integrate monitoring tools with data pipelines to continuously assess data against the defined quality metrics.
- Set up alerts and notifications for any anomalies or breaches in data quality standards.
Conduct Periodic Audits
Objective: Ensure long-term adherence to data quality standards.
- Schedule regular data audits, leveraging both automated tools and manual reviews.
- Compare the results against benchmarks or historical data to identify trends and patterns.
Feedback Loop for Issue Resolution
Objective: Address identified data quality issues promptly and prevent recurrence.
- Establish a clear protocol for reporting and resolving data quality issues.
- Collaborate with relevant teams (e.g., data engineering) to pinpoint the root causes of issues and implement fixes.
- Document resolved issues and lessons learned to refine the data quality framework.
Train and Educate
Objective: Enhance organizational understanding and capability regarding data quality.
- Conduct training sessions for teams involved in the data pipeline, emphasizing best practices.
- Share insights from audits and monitoring, promoting a culture of continuous learning and improvement.
Stay Updated with Compliance and Regulations
Objective: Ensure that data quality standards align with regulatory requirements.
- Continuously review industry-specific regulations and standards related to data.
- Update the data quality framework and practices accordingly to remain compliant.
Review and Refine
Objective: Evolve data quality practices based on feedback and changing organizational needs.
- Hold periodic review meetings with stakeholders to gather feedback on the effectiveness of data quality measures.
- Based on these insights, refine and update the data quality framework, tools, and procedures.
Document Everything
Objective: Maintain a clear record of data quality standards, procedures, and issues for transparency and future reference.
- Document the data quality framework, metrics, and procedures in a centralized repository.
- Record audit findings, resolved issues, and lessons learned.
If the data governance role or department follows this procedure diligently, they can effectively oversee and monitor the data quality in a data pipeline project. By doing so, the data remains consistent, accurate, and compliant with the standards set forth by the organization and regulatory authorities.
Image used under license from Shutterstock.com