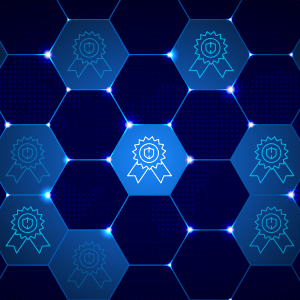
“Quality is never an accident. It is always the result of intelligent effort.” John Ruskin, prominent Victorian era social thinker
Data-driven decision-making is fast becoming a critical business strategy for organizations in every sector. However, if the data used to make these decisions is not high-quality, it can’t be trusted. And consequently, nor can the decisions that were made using it.
In this article, we will:
– Examine the importance of data quality
– Identify the factors that lead to poor quality data
– Present a case for employing a data governance manager
– Outline data quality best practices
Importance of Data Quality
Data is the fuel that propels massive projects of every sort, from important analytics drives to the introduction of company-wide artificial intelligence (AI) technologies. The measures put in place to ensure the quality of your data will determine the success of these initiatives.
Although data has been hailed as the new oil, data is like a crop when it comes to quality. Data is something that must be nourished at the source if it is to produce a good harvest.
Today, dedicated data scientists spend most of their time refining poor-quality data. It wastes a great deal of time and forces data teams to make assumptions about the data.
This can change the outcome of the data, but data scientists have no option but to make these hypotheses because the information they require isn’t there. This is why governance is so important in the data quality improvement process.
In fact, in a recent report, IBM crunched the numbers and concluded that a staggering $3.1 trillion is lost every year because of bad data in the US alone. There are a number of prominent examples where data quality issues have cost hundreds of millions of dollars, such as the numeric conversion error that led to the loss of a $125 million NASA Mars probe. So, data quality not only guides the success of major innovations, but the financial health of your company too.
Improving the quality of data is one of the most important objectives of data governance, but knowing how to do so is a significant challenge for most data governance teams. In general, data governance managers take a tactical and not strategic approach to addressing data quality problems.
Within every organization, individual users ordinarily focus their data quality concerns on the areas that affect them most significantly. Here’s a typical business scenario:
- A data governance manager may be interested in addressing the high-level fallout from widespread data quality issues.
- A project manager will be more concerned about issues directly affecting the success of their particular initiative.
- A CFO might focus on issues that directly affect shareholders, such as the ability to track losses.
By the time you finish reading this article, you will be equipped with the tools to develop a strategy for data quality improvement across your organization—regardless of conflicting priorities—and the knowledge to execute this strategy effectively.
Why Does the Data Quality Get Poor?
There are several related parameters that we can use to ascertain the quality of data. These include the data’s consistency, accuracy, completeness, and relevance (timeliness).
The most common reasons for poor quality data are problems with data collection in source systems and inefficient data analysis.
Working with Source Systems
When collecting data from source systems, a certain degree of standardization and defined controls must be in place. If you neglect to implement these measures, the quality of your data will suffer.
During the data capture phase: If you don’t prepare adequately for this initial phase, you will set the course for insufficient quality data set. For example, if you were to enter the customer’s phone number incorrectly, you might have difficulty confirming their identity further down the line.
Because of timeliness issues: The quality of data can depreciate over time, regardless of whether the data capture stage was successful. For example, a customer address may be recorded correctly at the initial capture stage, but if the address is changed and the information isn’t updated, there will be issues.
A lack of consistency due to inadequate standardization measures: If you capture data from different systems without using common standards, you will encounter inconsistencies. For example, when you capture measurement data from one system, you might encounter Kg or Km, while in the next, it might be recorded as Kilo or Kilometer.
All of these issues can be explained using the state code analogy. As you probably know, each state in the US can be abbreviated, and often is—it’s a lot easier to write MA multiple times than Massachusetts, for example. Many systems, such as payment systems, require users to enter their home state in capture forms.
However, if there are no predefined options in place and users can enter this information manually, you can be almost certain that it won’t match at all places.
Sticking with Massachusetts. Some users may choose to spell it out in full, others might abbreviate it to MA, while other users might choose to use MS or Mass. As a result, there will be multiple, conflicting codes for the same state in a single system.
The Data Analysis Phase
There are many ways that data quality can deplete during the analysis phase. For example, due to inaccurate field mapping, inconsistent formulas, or incorrect assumptions.
For data analysts to recognize the quality of the data in their organization, there must be specific processes in place. If these aren’t present, it can make data analysis very complex and convoluted.
As well as prohibiting effective data analysis, this lack of consistency also makes the merger and acquisition process very difficult. A key component of growth, when this digital transformation is disrupted, the fallout from bad quality data can be enormous.
In essence, poor quality data is untrustworthy, and as well as impacting company-wide initiatives, it will dissuade individual users from using it to innovate too.
Building a Case for a Data Governance Manager
One of the main stumbling blocks organizations come up against when it comes to improving data quality at a company-wide level is coordination. The trouble is, each individual employee has a different opinion on the data issues that exist and which are most important to them.
For example, an ETL developer is likely to judge data quality based on specific parameters. So, even if data quality is bad at the source, this isn’t something that is likely to affect the day-to-day running of their operations. However, if you ask the same question to someone responsible for maintaining a company’s CRM, they will be far more concerned with ensuring search terms match up in the system.
Because data quality issues aren’t limited to single applications, you need an independent, non-biased body or individual, like a data governance manager, to improve data quality across an organization. This independent body will essentially behave as a mediator, ensuring there are no conflicts of interest and roll out a data quality improvement strategy across an organization, crucially, in order of importance.
The next step is deploying a dedicated data governance suite. With a governance suite such as this in place, you can assure that everyone on your team is working from the same resource.
Introducing the Data Quality Improvement Lifecycle
Everyone in an organization believes their data quality problem is more important than everybody else’s. So, for a data governance manager or group to prioritize the importance of existing data quality issues, there needs to be a solid framework in place.
The primary use case of the framework is to discover which issue has the most business impact, as this establishes the order by which they will be addressed. This framework is the data quality improvement lifecycle, and it follows a delineated structure.
Define: The initial step in this process is to define your company’s data quality standards. This foundational step enables you to plan the direction of your data quality improvement program and set a series of core goals that you want to achieve.
Collect: The next step is to make a record of any data quality issues that exist in your organization. There are two key ways to do this. The first is to implement a data literacy program.
When most of your employees are data literate, you can introduce the next phase: installing a reporting mechanism where users can record data issues. You must record the following information:
- Business value
- The location of the problem
- What it is
- Priority
Prioritize: Next, you need to create a mechanism that enables you to calculate the business impact of the various data quality issues. This is a top priority for data governance managers who must consider the following:
- Business value
- Primary root cause analysis
- Anticipated effort to fix the problem
- Change management protocols
Using this process, a data governance team can ensure that issues are prioritized with maximum efficiency. However, if the team fails to come to a unanimous decision, which is quite often the case, there is a method to solve this: the introduction of a data governance committee. This committee must represent the whole company and be made up of different business leaders from across an organization.
This committee provides an additional level of appraisal and can consider issues, brought up by data governance managers, at a business level. Crucially, when data quality decisions are made that have implications across a business, there will inevitably be expenditure required to fund these changes. That’s why it’s essential that the committee is cross-departmental.
Analyze: After identification and prioritization, the member of staff responsible for dealing with the issue must conduct another root cause analysis. They will ask critical questions to determine how, why, and from where the issue arose.
Improve: Data quality issues can be fixed in four core ways:
Manually: Working with the source code directly.
In the ETL pipeline: Code can be developed that determines how the data is processed through your existing integrations. This is known as ETL logic.
Through individual process changes: It’s possible to change aspects of processes that encourage bad data quality. For example, asking users to select an option from a drop-down menu is far less risky than asking them to input values manually.
Through master data and reference data management: Data quality issues often present themselves when master data is absent. For example, mismatched data sets could arise when two systems hold the same information for a single customer but only the email is correct in both.
One solution is to create a single location to store all master data. This way, multiple systems can reference it using keys. Although often expensive and always complex, master data management is very efficient.
Reference data usually takes the form of lists that can be referenced by master data and are static. Access controls and relationship mapping can help to manage this reference data.
Control: Finally, you need to create a set of data quality rules. These will ensure that the issues you have uncovered won’t affect your organization again. If they do arise, users will be automatically notified.