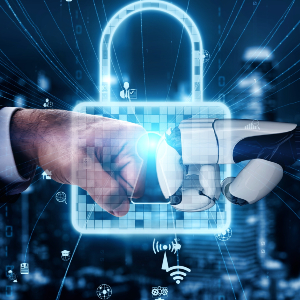
Data privacy and security matter. Given this, it will be a big deal if machine learning can make them easier to implement. So, how do CIOs and other security thought leaders think machine learning applies to privacy and security? Let’s start by looking at the risks and then discuss how technology makes addressing them easier.
Largest Privacy & Security Risks
So, how are privacy and security risks perceived? What are the biggest risks for enterprises? Is there an owner for addressing these risks? And just as important, do they have the budget and authority to address them? I asked several CIOs to weigh in during a recent conversation.
Interim CIO and consultant, Anthony McMahon, said “I will come out and say it. It’s people. Additionally, organizations tend to not invest enough on data security. It’s a decision when an organization invests or does not invest the right amount.”
Analyst Jack Gold agrees by saying, “the single biggest security risk remains not having all employees on-board with the security plan and training for that plan. Technology is not enough, but it is needed as you can’t eliminate all human error. This is where ‘Zero Trust’ wants to play, but it still has a long way to go. The owner should be the C-Suite.”
Former CIO Isaac Sacolick interestingly believes “the biggest privacy and security risk occurs when there isn’t an owner with authority or budget. And unfortunately, there is no magic wand to fix this.” In terms of what are the risks beyond people and investment, Constellation Research Analyst, Dion Hinchcliffe says, “the large privacy and security risks for most boil down to:
- Cybersecurity breaches
- Insider threats
- Inadvertent data leaks or spills
- Misused data in second order consumption
- IT and digital departments (i.e., marketing and digital customer experience)
An interesting question is whether the security team should also be the privacy team. Obviously, there will be overlaps. But digital privacy should also be the responsibility of compliance, legal, DevOps, and even enterprise architecture.” Hinchcliffe continued by saying, “DevOps makes sense for these areas. But then you realize if you want to be security first and not try to graft it on later, you need DevSecOps. At the same time, cybersecurity is not an enabler of application development. It’s a way to make it slow down. We need for this reason to make security intrinsic.”
Impact of Standards
Next, I inquired about the importance of deploying standards and whether organizations have adopted the principles including ISO, NIST, and ‘Privacy by Design’. McMahon said that one of the organizations he worked with in the past was heavily influenced by NIST and two others had ISO 27001 guide them. Many organizations are now ISO and NIST certified, although they typically vary in the degrees of compliance. Few have gone down the ‘Privacy by Design’ road yet, and HIPAA, PCIDSS, CCPA, and GDPR remain the most impactful.
Miami University CIO David Seidl relayed that he is open to, but has not implemented standards yet. He says, “privacy is something I think will need to focus on for organizations of all types. As usual, I’ll bang my drum about the lack of national level privacy legislation. The piecemeal efforts at state levels is chaotic and creates compliance nightmares.”
Without question, as AI proliferates, new standards will become a necessity. A recent HBR article, AI Regulation is Coming, says, “AI increases the potential scale of bias: Any flaw could affect millions of people, exposing companies to class-action lawsuits.” This means that organizations will need to not only control private information, but also control the application of private information into data models. As data is provisioned, access to machine learning models of data that can lead to biased outcomes must be controlled. In a personal conversation with Tom Davenport, he stated that “bias comes from data not models.”
Biggest Opportunities for Machine Learning in Privacy and Security?
CIOs had several thoughts about the potential. These included:
- Anomaly/fraud detection
- Predictive analytics
- Proactive measure to potential threats
- Analyzing content for confidential data and taking actions on them to prevent accidental/intentional leakage
- Automated analysis of software and code in development pipelines with coaching to improve security
Before sharing on the opportunities, Hinchcliffe stressed that, “there are AI threats and risks. Worse yet, I think there are several reasons CISOs can’t be more proactive about these:
- Intense daily operational distractions
- A belief that security/privacy standards are an enormous industry-scale undertaking.
- A belief that Zero Trust is the big focus and will take years to realize.”
Part of the issue for CISOs and their CIOs is how big cybersecurity has become as a domain, never mind privacy. Hinchcliffe’s latest maturity model for cybersecurity demonstrates. There is so much to do.
Hinchcliffe continued by saying, “we must make security intrinsic. The biggest opportunities for ML is privacy and security to do the following:
- Creating clear org-wide policies
- Making them machine readable
- Mandating MOPs and ModelOps as the way to consistently build and run compliant ML models
- Automate verification of GRC across all ML ops
- A system of systems approach
Yet, it is critical that everyone remember that Cybersecurity and privacy are related, but different.”
Could Machine Learning Eliminate Risks by Holistically Discovering Exposed Data Risks?
Hinchcliffe says, “I dare say only automation will be ultimately able to secure IT. We must shift to a holistic ML approach to static and dynamic threat analysis to reduce risk. AI will also help bad actors find exploits and white hats to fix them first hopefully.” However, Seidl adds that, “they’re not going to cover the entire space. They may be part of your controls. The next round after this will target the AI to see how they break in interesting ways.”
Balaji Ganesan, CEO of Privacera, says, “Dion Hinchcliffe is correct. AI can help and hurt. For this reason, we see our customers using AI and Machine Learning to drive what the authors of the “Privacy Engineer’s Manifesto” call the ‘intelligence stage’ of data security and privacy. Here, AI and ML find sensitive data and then use this to ensure that access is granted intelligently, including noticing improper or unnatural accessing of data. It can also deal with model bias by limiting data models access to data that can lead to bias.”
Parting Words
It seems clear that security and privacy is growing in importance for organizations. While ML offers the potential to make things easier to implement, it also creates risks. The bad folks will get more sophisticated in a never-ending arms race. With this said, maturity matters. In the words of the authors of Competing in the Age of AI, “we need to build a secure, centralized system for careful data security and governance, defining appropriate checks and balances on access and usage, inventorying the assets carefully, and providing all stakeholders with the necessary protection.” We need to finally drive ethical AI by controlling model access to sensitive data as well. Daniel Yankelovich was right when he wrote“New Criteria for Marketing Segmentation. Here, he said that demographics are not an effective basis for marketing segmentation. Doing this will prevent bias and privacy loss in the application of data.