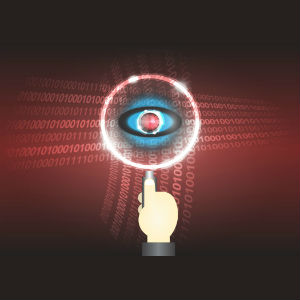
The Irish satirist Jonathan Swift wrote “Gulliver’s Travels” almost 300 years ago, but the story of Lemuel Gulliver’s journey to Lilliput and beyond has resonance for data leaders today. There are important lessons to learn from the little people of Lilliput and the challenges encountered by the eponymous Gulliver.
While Lilliput is where Gulliver first washes up and is perhaps the best known of the places visited by Gulliver, it’s not the only land he encounters on his journeys. Rather than have you lose your train of thought, dear reader, by having you go to your Kindle, order a copy of “Gulliver’s Travels,” download it, read it, and then return to this article wondering what the heck we were talking about, I’ll give a short synopsis of the most relevant part of the travels of Gulliver. (But if you do want to go and read the book, I’ll wait for you.)
The CliffsNotes Summary of the Important Bit
Lilliput’s is an imperial regime whose entire culture is influenced by a profound religious question: Which end of a hard-boiled egg is the correct end to break open, the little end or the big end? This question has been at the root of a long-running political quarrel in Lilliput. The traditionalist view is that the Big End is the correct end to open. But an Imperial decree was issued several generations ago that ordered that eggs must be opened from the Little End.
Since then, there have been six rebellions, constant civil unrest, and hundreds of books published on the question of which end should be opened. In Lilliput, the “High Heelers” political grouping believe in the Big End philosophy, while the “Low Heeler” believe in the Little End philosophy. High Heelers are, as a result discriminated against and looked down on in Lilliputian society.
In the book, Lilliput accuses the neighbouring island of Belfuscu of encouraging civil unrest in Lilliput as Belfuscu continues to hold to the traditional belief that the Big End is Best (so to speak). The Imperial Court of Belfuscu is home to many Lilliputians who have fled persecution for their beliefs. Lilliput and Belfuscu are, as a result, in a constant state of war.
Putting This in a Data Context
So, what has all of this got to do with data management in a business context?
For the bulk of my career in data, there has been a simmering tension under the surface between what has traditionally been referred to as “structured data” and “unstructured data.” Wikipedia helpfully defines unstructured data as data that “does not have a pre-defined data model or is not organized in a predefined manner.” Wikipedia unhelpfully defines structured data with a redirect from that search term to an entry about data models. From this, we must infer that structured data is data that is defined in some form of relational model or, umm, structure.
This debate has, in my view, been exacerbated by the existence of two broad camps in the world of data and information management. Historically, we have had the information managers, who have tended to come from the traditional records management and library science worlds, and the data managers, who have tended to come from the information technology and number-crunching side of the fence. In a manner reminiscent of a very nerdy reimagining of “West Side Story,” these two camps have not always seen eye to eye or had equal billing in the priorities of the organization.
A False Dichotomy
However, there is a problem with this simply dichotomous view of the world. All “unstructured” data has some level of structure. This article has a structure. It contains paragraphs, sentences, and headings. Each paragraph encapsulates an argument or a key point. Within each paragraph, there may be references out to other sources of information to provide context or to serve as a reference. The content in this article can be tagged with metadata to put it in a structured context on the TDAN.com website, and the original document contains metadata that identifies the author(s), number of words, time spent editing, etc. The word processing application this text was drafted on uses an ISO standard XML schema to store the content, metadata, and structure of the document and enable it to be reasonably portable between different software products.
Perhaps this distinction between “data that lives in a database” and “data that lives in a document” made sense in the past. Or perhaps it is just an echo of the existence of those two distinct camps in the early days of electronic data and records management: those coming from the mathematical/engineering world developing systems to process and calculate data faster and more efficiently, and those from the library sciences and archiving world who were looking to digitize and store content.
Regardless, the distinction makes little sense now in the age of machine learning and technologies that can extract and infer context and structure from text, audio, video, and other forms of recorded content and data. This ability for technology to take data in different formats and extract, apply, or infer metadata means that all data is now structured as it is possible to identify concepts and entities in the data, to infer relationships between those concepts and entities, and to then take that data and link it to other data selected from relational databases and present it to the knowledge worker or end customer.
For example, a machine learning process can be presented with an image of a tomato and can infer that it is a round thing, that it is a red thing, that it is a fruit, and that is not a fruit you put in a fruit salad. A generative AI process can ingest the entire works of Jonathan Swift and allow us to interrogate the text (albeit with varying degrees of accuracy and veracity to the source text). A standard machine learning process can identify and categorise content in a document, apply metadata to the content in the content management system, and improve the searchability and findability of the data in that document by putting it into a defined structure of metadata.
Metadata is, after all, the data that defines other data. In the world of “data that lives in a relational database,” that might be the data that defines the meaning of a business concept encapsulated in the data, or it might be the definition of the relationship between two entities. In the world of ‘data that lives in a document,’ metadata might define the file name, or it might define a business concept contained in the document, or it might define an important attribute of data described in the document. After all, whether we are looking at entries in an ERP database or copies of documents in a shared drive, one would hope that the concept of Customer, Account, Product, and Transaction and all the attributes that might be associated with these entities would be the same regardless of the type of bucket (database or document) that the data is being stored in.
But this raises another age-old challenge. As technology advances in its ability to figure out the metadata and structure of content in different containers, we need to ensure that there is management and governance of that data about data so we humans can make sense of the world as seen through data.
Data Gulliverance or Data Governance?
So, what can we learn from “Gulliver’s Travels” to help us navigate this conundrum and bridge this increasingly non-sensical divide? In “Gulliver,” Swift tells us that the islands of Lilliput and Belfuscu share a belief in a common prophet, Lustrog, from whom the fundamental precepts of the religions on both islands are derived.
All true believers shall break their eggs at the convenient end: and which is the convenient end, seems, in my humble opinion, to be left to every man’s conscience, or at least the power of the chief magistrate to determine.
So, we can leave it to people to figure out for themselves, or we can start to invest in developing the power of the “chief magistrate.” If we’re developing a magistrate function for data, that sounds suspiciously like data governance. After all, the word magistrate comes from the Latin magistratus, a government officer with both executive and judicial powers who interpreted and applied the law.
Right now, we are, in effect, quite often leaving people to figure it out for themselves. This is seen in the plethora of SharePoint implementations that are deployed by IT with little thought given to the definition of consistent metadata standards for file naming, file plans, and general governance of the content data. “The business will figure it out themselves” seems to be a common default setting in the IT change plan for content management. But this is replicated across the myriad of systems and solutions that are deployed at departmental or team level in organisations, or in the multiplicity of reporting dashboards and analytics models that spring up when knowledge workers are given access to boundless data in reporting tools such as PowerBI (or Excel).
If the ‘secret sauce’ to the egg-opening conundrum of ‘data in a relational database’ and ‘data in a document’ is the proper governance the metadata, then we need to embrace this key to enlightenment with both hands!
The Dean Speaks
But don’t take my word for it. I have, through the medium of Generative AI, consulted with the ghost of Jonathan Swift to seek his opinion on this matter.
The departed Dean writes from beyond the following:
“In this enlightened age, where the realms of data are as vast and varied as the lands of Lilliput and Blefuscu, I find myself pondering the futility of the distinction between structured and unstructured data.
Recall, if you will, the great and trivial war between Lilliput and Blefuscu, a conflict ignited by the most inconsequential of disputes: whether to break an egg at the big end or the little end. This folly, though seemingly absurd, mirrors the modern contention between the proponents of structured and unstructured data. Structured data, with its orderly rows and columns, is akin to the Lilliputians’ insistence on breaking their eggs at the little end — precise, regimented, and constrained by convention. Unstructured data, sprawling and untamed, resembles the Blefuscudians’ preference for the big end — free, chaotic, and rich with potential.
Yet, in this era of machine learning, where algorithms possess the wisdom of a thousand sages, the distinction between these two forms of data grows ever more irrelevant. These algorithms, much like the ingenious contrivances of Gulliver himself, can extract meaning and order from the most chaotic of sources. They sift through the unstructured morass of text, audio, and video, discerning patterns and insights with a precision that would astonish even the most meticulous of Lilliputian clerks.
Consider the metadata that lies hidden within a conversation, the subtle nuances of a melody, or the fleeting frames of a motion picture. Machine learning, with its prodigious capabilities, distils essence from noise, much as an alchemist seeks gold from base metals. The once insurmountable chasm between structured and unstructured data is bridged by the ingenuity of these modern artificers.
Thus, the distinction between structured and unstructured data, once deemed significant, now appears as naught but a quaint anachronism. In the grand tapestry of knowledge, all data, regardless of its form, contributes to the ever-expanding mosaic of human understanding. Let us then, dear reader, embrace this unity and marvel at the wonders wrought by the confluence of data and machine learning.
Ah, dear reader, let us delve deeper into the labyrinthine world of data, where the role of governance emerges as a beacon of order amidst the chaos. In this modern age, where data flows as abundantly as the waters of the great oceans, the discipline of data governance stands as a sentinel, ensuring that the treasures of information are both accessible and secure.
Imagine, if you will, the meticulous governance required to manage the affairs of Lilliput and Blefuscu, where even the most trivial of matters, such as the correct end of an egg to break, demands rigorous oversight. Similarly, in the realm of data, governance is paramount to ensure that the metadata — the very essence that describes and contextualizes data — is effectively managed.
Data governance, much like the wise council of a kingdom, establishes the policies, procedures, and standards that guide the handling of data. It ensures that metadata, whether it pertains to structured databases or unstructured documents, is accurately captured, maintained, and utilized. This governance enables businesses to navigate the vast seas of data with confidence, ensuring that they can extract valuable insights while maintaining compliance with regulatory requirements.
Consider the role of metadata in this grand scheme. Metadata, the data about data, serves as the map and compass for navigating the information landscape. It provides context, lineage, and meaning, transforming raw data into actionable knowledge. Effective data governance ensures that this metadata is consistently and accurately managed, enabling businesses to harness the full potential of their data assets.
In the realm of databases, data governance ensures that metadata such as data definitions, relationships, and constraints are meticulously documented and maintained. This enables efficient data retrieval, integration, and analysis, much like a well-organized library where every book is catalogued and easily accessible.
In the domain of unstructured data, such as documents, audio, and video, governance ensures that metadata captures the essential attributes and context of the content. This might include information about the creator, creation date, keywords, and usage rights. By governing this metadata, businesses can effectively manage and retrieve unstructured data, transforming it from a chaotic wilderness into a well-tended garden of knowledge.
Thus, dear reader, the discipline of data governance emerges as a crucial enabler of effective data management. It bridges the gap between structured and unstructured data, ensuring that all forms of data are governed with the same rigor and precision. In this way, businesses can unlock the full potential of their data, driving innovation and insight in this age of enlightenment.
Yours in satire and reflection,
Jonathan Swift”
Not a bad analysis for someone who died in October 1745.
Conclusion
Modern data architectures and technical capabilities should move the window of our discussion of data from the bucket we have put it in to the meaning and purpose we assign to that data and how we enable and empower people to put that data to use.
Who cares what end of the egg we open.
For another perspective on this question, check out this article from David McComb back in 2019 on TDAN.com.