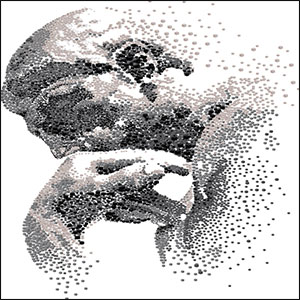
This column is next in the series of how organizations achieve and sustain significant improvements in fundamental data management disciplines in their journey to Great Data. Content and conclusions are based on my work with many organizations to evaluate, accelerate and enhance their EDM Programs.
We’re going to explore “What Good Looks Like” for the Business Glossary, summarizing the collective accomplishments of high-capability organizations, with examples of benefits and challenges overcome. (Names withheld to protect the superstars from the paparazzi). We’ll also provide suggested approaches, key implementation steps and indicate the work products you need to help your organization follow in their footsteps.
Data Managed as Meaning
Data problems are business problems. This is the “so what?” of the Business Glossary. It’s as simple as that.
Therefore, there is nothing, nothing, nothing (I say unto you once more, nothing) more fundamental to effective data management than the business glossary, a compendium of harmonized and approved business terms that unambiguously name and define shared business concepts. Data meaning is the sine qua non of all data management disciplines, and the central core of the organization’s data knowledge.
A Business Glossary is a compendium of approved and managed business terms. It is the mechanism by which formalized agreements about the meaning of key concepts are captured, and common terminology developed that is understood and shared across business lines.
Varying usage of business terms across an organization is essentially a business problem – a lack of essential communication which becomes concretized in application data stores, perpetuated in repositories, and causes discrepancies and inaccuracies in reporting. Multiple definitions, interpretations, and uses of the same terms in different ways, contributing to an ever-growing complexity of mappings, reporting challenges, and misunderstandings about data, which negatively impacts data quality and reliability.
Divergent understanding and use of business terms show up in the classic problems of concepts sharing the same name, but implying different meanings for varying users, as well as the same concepts given different names. These discrepancies cause confusion, delays (and at worst, avoidable halts in the action and bad business decisions). They typically surface as an issue when attempting to integrate systems containing the same (or overlapping) data sets, resulting in errors that increase rework and undermine confidence in aggregated information. Business and technical users may need to guess whether a term name refers to their typical business usage, or has another (broader, narrower, or different) meaning. This ultimately breeds an overall lack of trust in the data.
The hub and spoke diagram below shows the centrality of the Business Glossary and its relationship to other DMM process areas.[1]
Capable organizations understand that consistent use of shared business terms solves many business problems. If your organization hasn’t paid attention to developing and approving shared business terms, you can experience a world of hurt.
For instance, an insurance company experienced many issues because its federated business areas did not consistently define or distinguish between ‘Program’ and ‘Product.’ This resulted in:
- Painstaking and extensive manual effort to integrate global financial results
- Many reporting errors
- Lack of communication and agreement among business executives
- Fuzziness in the organization’s business strategy, and
- Impact on the accurate assessment of exposure to risk.
That was the ultimate problem, because as we know, the ‘Art of Insurance Risk Exposure Estimating,’ from the company’s point of view, can be rendered, in sum, as “How much do we need in cash reserves?” Mistakes here can lead to serious financial situations, affecting credit ratings and the ability to pay claims (and thus reputational demerits and competitive disadvantages, etc.).
Another example illustrating the importance of agreement about business concepts – a State agency had been formed from many smaller agencies. Several years into the consolidated organization, they were still unable to count the number of individuals served. The root cause was a lack of agreement about the definition of ‘Client’ (in general, a person receiving one or more of their services, or required to pay fees, or to carry out mandated actions) across departments. This led to big challenges in data integration, and affected funding, budgeting, staffing, and reporting to the legislature and Governor. It also led to unfavorable audits and a host of other persistent issues (e.g., deriving householding, a big challenge).
[For more examples, Business Glossary concepts, and relationship to other DM disciplines, please refer to a previous column “Evolving the Business Tribe: Centrality of the Business Glossary.”]
Let’s jump into what GOOD looks like, distilled from a number of organizations who have implemented well-integrated and comprehensive EDM programs, and as a measure of their success in managing data as meaning, have achieved high scores in the Data Management Maturity (DMM)SM Model’s Business Glossary process area.[2]
Notable Accomplishments
These are the achievements that strong organizations have typically implemented:
- A defined Business Glossary process – addresses what the activity steps are for how terms are scoped, selected, defined, approved, modified and published, with corresponding roles and authorities
- Business Term Standards – defines theproperties of business terms and how they should be captured and managed, provides guidelines for naming, definitions, and other properties, like domain stewards and statuses, and specifies approval and change procedures
- A Business Glossary Policy – addresses the conditions under which terms must be defined, how they shall be used, and by what roles / organizational units, e.g., for forward design, for regulatory reporting, for data requirement specification, etc. Specifies the compliance process, i.e., how the organization will assure that the policy is being followed.[3]
- Business terms defined for core shared data domains – a significant portion of the organization’s enterprise data is defined and approvedaccording to the process, and employed according to the policy
- Glossary published and easily accessible – business termsarecommunicated, published, and made available in a centralized online location
- A plan for populating the Business Glossary – aligned with the Data Management Strategy[4] and prioritized domains, including in-flight and planned efforts involved shared enterprise data
- Traceability to operational and technical metadata, data designs, repositories, data stores, APIs, and interfaces- business terms are the starting point for business metadata, which may include dates, statuses, approval levels, specification of allowed values and ranges, etc.
- Active, engaged data governance – organized, efficient, active participation by data stewards and business data experts, stakeholders knowledgeable about the scope of the phased Business Glossary effort, to develop crisp definitions, coordinate, resolve conflicts about naming and definitions, and approve terms
The last bullet could be bold, underlined, and in a 20 point font, without any exaggeration. There is a story about a desert shaikh who was teaching his son how to select a horse, essential for both transport and defence. He placed a horse behind the tent flap lifted it up slightly, so only the hooves could be seen. His son asked, “Why are you not showing me the whole animal?” And the shaikh answered “Because, my son, without strong sound hooves, your feet will be scorched by the burning sands.” And the motto is: “No hoof, no horse.” (And the parallel is “no governance, no glossary,” true for every organization).
What benefits do organizations realize from implementing these capabilities? Well, at a minimum:
- Improved communication about key concepts across the organization, leading to better business decisions
- Effort and cost savings realized from applying approved business terms to project scoping efforts – aka, not reinventing the wheel
- A head-start on conceptual and logical data modelling efforts, avoiding increasing complexity
- A solid foundation for quicker data integration and a template for APIs and views
- Improved speed to insight for analytics teams as they integrate data for reporting and modelling
- Future cost savings by enabling domain alignment, data store rationalization, consolidation and migration, tracing data lineage and supporting the reuse of quality rules for shared data
- Substantiation for regulators that the organization is taking practical steps towards managing its data efficiently and accurately.
In short, this is an effort well worth undertaking, and there is really NO adequate replacement for it. (So, stop complaining, bite the bullet and start somewhere, using the tips below).
Scoping and Where to Begin
Overall, the objective of uniquely defining business terms for shared enterprise data is best approached as a phased effort, utilizing the impetus of specific priority initiatives. By following a plan organized by data domain, set out in the Data Management Strategy (combined with the added urgency of key planned projects), the organization will eventually achieve a well-populated business glossary for all enterprise data. This may take a few years because many organizations have thousands and thousands of business terms. Not even an omniscient being can know all of them a priori.[5]
Hence, the business glossary should be developed as a part of other activities that are closely linked to business benefits. If you build out a business glossary as a stand-alone parallel effort without direct incremental benefits, such as improving business processes and supporting implementation projects, that effort will be short-lived and may lose funding if near-term value is not demonstrated.
Some tips about scoping. Pick an upcoming priority project – for example, a new master data store, a new data lake,[6] adding new data to a data warehouse, a custom-to-vendor product migration, etc. Align the data scope of that effort to data domain priorities (often associated with major programs or projects) that were documented in the Data Management Strategy.[7] That puts you on the enterprise data path, with no loss of time to delivery. (A win-win).
With reference to scoping by domain, if your organization doesn’t have a Data Management Strategy,[8] it can be simplified by applying the layers shown in the diagram, in sequence.[9]
Now that you’ve defined scope, what activities are you going to undertake? First, think about your approach to balance the factors that will affect speed, quality, and completeness. I recommend a multi-pronged approach – a judicious combination of these three avenues: Top-down, middle-in, and bottom-up.
- Top-down – the organization determines the initial priority set of business terms, such as highly shared data, data critical to core business processes, or data required for regulatory reporting. This is a task for governance groups, requiring discussion, review, qualification (i.e., renaming terms, or adding one or more name components for precision), and approvals. It is linked to the data domains that were (again – we hope) defined in the Data Management Strategy. For truly fundamental business concepts for the organization, the top-down approach should be applied for critical data elements – e.g., Client, Product, or Loan master data, etc., etc.
- Middle-In – the Data Management Function (centralized or distributed)[10] in collaboration with data governance, gathers business terms from corporate policies, external standard terms that require adherence, the lines of business, Human Resources, Finance, Risk, and other organizational units that have probably developed clear business terms. These can be parsed and reconciled with both top-down and bottom-up lists of terms; following the middle-in approach, one frequently discovers terms that have different names but the same meaning, or the same meaning with different names. ‘Beating the bushes’ for existing terms is typically quite fruitful – why think through all of them from scratch versus leveraging previous efforts?
- Bottom-Up – the Data Management Function, in collaboration with established governance bodies or a data working group, can discover candidate business terms from operational data store and repository data dictionaries, creating the initial terms based on the physical data elements. This effort will be required to discover additional business terms for parsing and harmonizing with the Top-Down and Middle-In results. The probability is, relative to the Middle-In avenue, that there will be even more divergence in term names, definitions and allowed values.In addition, you will need the cooperation of operations and maintenance project teams (no help for it, data call time).The advantage of following this aspect of the approach is completeness – you will eventually discover every grain of wheat, grist for the Glossary mill.However, the engagement of data governance is just as important – you can’t really skip any key steps for evolving a Business Glossary, you can just change the order of operations to harmonize with current circumstances, resources and priorities.
In sum, consider the overall approach in the light of this question: “How do we find all the terms that need to be defined?” For large organizations with tons of data stores and few defined and approved business terms, the Bottom-Up and Middle-In paths will yield the greatest number of candidate terms more quickly. For instance, if the organization’s objective is to consolidate data stores or create authoritative repositories for a data lake, the easiest path would be to comb the universe of data stores aligned to domains first, yielding the macro-set. Then, aftter identifying redundant or overlapping data sets, hone in on the areas of greatest concern, a chunk at a time.[11]
Business Glossary Development
In planning for business term definition and approvals, these are the core elements to explore and address in the plan, and corresponding work products to produce for a consistent and successful result.
- Define and adopt business term standards – a template for business terms, definitions, and associated business metadata, which can be leveraged for all programs and projects that depend on shared data.
- Define the Business Glossary process – describe the process of proposing, reviewing, defining and approving business terms. Using the standards, specify inputs, outputs, controls, mechanisms, roles, approval authorities, and escalation steps. Then, augment the process with how terms can be modified and how modifications and additions are approved. My recommendation is to spend as much time on this activity as you need, because you want all stakeholders to agree. For that to occur, they must be involved in the process definition and description and concur with the activity steps.[12]
- Educate active participants – develop an educational presentation with examples from your own organization, or purchase computer-based training and couple it with your standards, process description, roles, etc.
- Research applicable external standards – for some industries, widely accepted standard business terms exist, for example the Mortgage Industry Standards Maintenance Organization (MISMO) for exchanging information and conducting business in the mortgage finance industry. If they exist for your industry and selected scope of work, start there – you can either adopt them as-is or further qualify them (e.g., refine the definitions by adding explanatory text on usage after the core definition, or adding name components as needed to correlate with the enhanced definition).
- Use well-known and respected industry dictionaries – of the best-selling and most widely used products available, select a starter set that your working group will start with as baseline definitions. For example, at the Nasdaq in the 90s, everyone defining terms and engaged in data modelling efforts had a copy ofBarron’s Dictionary of Finance and Investment Terms. Using it as a baseline allowed everyone to begin from the same concept description, cleared up a lot of confusion, and prevented many potential disagreements.
- Decide whatgeneral dictionaries you’ll use, if there is no industry-wide dictionary available – will it be the Oxford English Dictionary, Merriam-Webster, American Heritage, the Google Dictionary? Or all of them in a specific order? Stating this up front will help with the baseline definition discussions
- And then,apply the art of definition – if a business term is quite specific to your organization, or not found in any of the standard sources, your working group has the excitement of creation. This is where the discussions get philosophical, since after all, a definition is an attempt to render in words the essence of the Thing-in-Itself. (For example, is a motorized skateboard a ‘vehicle?’)
A big bonus of this approach is that your process and work products will be highly reusable for all business areas of the organization. The Business Glossary is actively taking shape and being populated, and the corresponding work products can be applied across the organization. Ideally, the glossary may start in a spreadsheet, but will end up in a metadata repository, where the terms will anchor all other metadata (attributes, physical data elements, datatypes, and the huge sweep of operational, technical and process information). This will allow robust search functionality and make the terms available to all stakeholders.
Defining business terms is the consummate data governance activity. If led effectively, it is fun, it is meaningful, and engenders a sense of accomplishment. When a group has successfully standardized, defined, and approved shared business terms, they will gain in knowledge and confidence for the next tranche of terms. The process will soon become ‘Business as Usual’ and gradually raise the level of data awareness and governance engagement across the organization. The same approach can be applied for defining the meaning of aggregated and calculated terms used in standard reports, trend discovery, and predictive modelling.
In
terms of value – when conducting vendor data feed evaluations and selection,
you’ll probably find that they provide detailed business term definitions for
their products, sometimes in the context of a logical data model depicting data
relationships. This is quite helpful for the prospective customer, to fully
understand what they’re offering, and easily compare it to the organization’s
data requirements. They also often describe the business processes that are
followed to produce the data, and also the quality rules that are
applied. They go to these lengths to increase sales and customer confidence,
but if you look at it from the perspective of your organization, thinking long
term, your business terms are the core kernels of business knowledge. Why not aim
for the best?So happy defining! In the next column, we’ll share best practices
from strong organizations in Communications, Business Case, and Funding. And
[1] Relationships depicted in the diagram are defined and described in a previous column “Evolving the Business Tribe: the Centrality of the Business Glossary.”
[2] The highest score achieved to date in my 30+ EDM Assessments has been 3.75, and the lowest, 0.50 on the DMM’s Five Level scale. (Level 3 is organization-wide, green light, good, go, recommended for all organizations).
[3] One example of a policy topic – business terms should be required as part of the data requirements definition process to support new development or integration efforts. Also applicable for implementing for vendor system products since they contain proprietary screen and field names that often differ from the language of the business. Therefore, prior to converting a custom data store to a vendor platform, an organization should define the business terms currently instantiated in the source system(s), and then map them to the new platform. (E.g., Client Status = Flexfield 24 in the CUST_STS_HST table).
[4] See column “Almost Heaven Part 1 – Enterprise Data and the EDM Strategy” – bottom line, you need to define ‘enterprise data’ and create a Data Management Strategy.
[5] This is where an external regulator can be your best friend. One bank was able to define over 2,000 terms in six months (well-resources, of course) with the scope defined by regulatory reports. Coupled with other successful EDM efforts, they achieved their first Satisfactory audit. Yay!
[6] For example, a frequently encountered situation is an internal business customer that wants to add new data to an existing data lake. You can add business term definition to the front end of the onboarding process / policy.
[7] See? Emphasizing the point one more time – you really do need a Data Management Strategy to orient prioritization of focus for implementation, not just overall, but also in the context of most DMM process areas. You can’t build the Golden Gate bridge in a week.
[8] At this rate, we’ll soon be able to chant “shame, shame” in the halls.
[9] See “Almost Heaven – Enterprise Data and the Data Management Strategy “for a description of these layers.
[10] See the DMM’s Data Management Function (DMF) for purpose, goals and functional practices, and my column “The Perennial Question” which distinguishes and addresses collaboration between data governance and the DMF.
[11] How many? Count on 5-10 terms per group hour, more if they are non-controversial, less if you hit a snag
[12] This is a terrific group exercise. When I take a sample data management process and work with course attendees to model it together, the outcome is always positive. Everyone is interested and contributes their opinion and rationale. Governance at work!