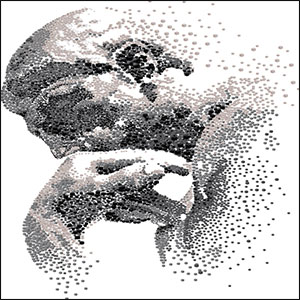
Some countries successfully create long-term strategic plans. For example, China’s first 100-year plan was aimed at the elimination of extreme poverty by 2020. In 1980, there were 540M people living in extreme poverty; by 2014, there were only 80 million. The second 100-year plan, targeted for 2050, calls for achieving 30% of global GDP, to transition to some form of democracy, and to clean up the environment. In 1980, China’s GDP was 1% of the world GDP; by 2015, the GDP was 15%.
When coupled with the will to execute, and supported by commensurate funding, direction, and governance, strategic planning works. We’ll explore this further relative to the EDM function.[1]
My last TDAN.com column, Failing to Plan is Planning to Fail, included the following chart created by Delorese Ambrose, a professor at Carnegie Mellon. In that column, we discussed the factors that need to be in place to effect positive change in the EDM function and outlined key topics that should be included for important EDM plans, including an EDM Strategy, a Metadata Strategy, a Communications Strategy, and governance charters.
We stated that for an EDM Strategy, we needed to first decide ‘What Good Looks Like’ at the macro level of the EDM Function. For this column, we’re going to examine typical obstacles to envisioning what The ‘Good’ may be for your organization. Looking at the same diagram, note in the red circles that the lack of Vision is the primary cause of Confusion, because there is no mutually agreed answer to “Where are we going?”
As I continue my work with organizations to evaluate their EDM functions, assist them in determining their implementation roadmap and deciding what is most important to accomplish, an underlying theme is to help them gain clarity, developa vision and thereby reduce confusion – aka, if you don’t know where you’re going, you can’t plan the trip, arrange transportation and accommodations, and pack the right clothing. I’ve observed that organizations which haven’t yet succeeded at crafting an organization-wide vision and strategy for EDM frequently exhibit many of these characteristics:
- Lack of an articulated business strategy – organization goals and concrete objectives to achieve these goals
- Difficulty understanding that business problems are often data problems – e.g.,no agreement on sharedkey concepts (business terms), no focus on metadata, no organization-wide approach to improving data quality, etc.
- Lack of a clear scope – aka, no clear definition of what constitutes ‘enterprise data’
- Distributed culture and organization – aka, primarily conceiving and funding data initiatives in silos for specific line of business purposes
- Lack of operationalized data governance – no executive governance group, no designated data owners
- Lack of a centralized EDM organization – aka, no backbone or shepherd capability for persistent data management products
- Lack of organization-wide functions – aka, no organizational unit focused on enterprise architecture, no organizational unity focused on business process improvement, no organizational unit focused on the target data architecture, etc.
- Lack of executive commitment to managing data as a critical asset.
Obviously, these fundamental issues will inhibit the ability of the organization to craft an enterprise-wide vision for EDM excellence. Let’s analyze these obstacles a bit further.
Anarticulated Business Strategy should be determined by senior executives and the Board of Directors, starting with an organization vision and broad goals. It is beneficial to set goals and objectives for varying timeframes, e.g., a 5 to 10-year strategy, a 3-year strategy, and a 1-year strategy. Many organizations of all sizes do not publish a business strategy – however, they may have one, but it is closely held, because they don’t want competitors to learn about it.
Let’s say the business Vision is “To provide safe, electric self-driving passenger cars at a fair price for US and global consumers.” The vision statement is like a function description, a brief answer to the question “Who are we?” and “If we’re being who we are, this is, in a nutshell, what we do.” Then the organization would determine its business goals for the strategy timeframe addressed, for instance, for a 5-year strategy “Expand our market share in Europe by opening a factory in France in 2023, to comply with EU manufacturing standards.” Next would be the objectives – specific actions that support achievement of the goal – for instance, they might include: “Finalize land purchase by December 2019;” “Determine market requirements for EU electric vehicles for 2024 models;” “Review and approve factory design by May 2020;” and “Unveil Proof of Concept vehicle at the 2023 International Auto Show.”
Achieving these objectives will require execution of business process activities and imply data requirements. So for the “determine market requirements for EU electric vehicles,” there would be a research effort that would likely look at: current standards; EU customer preference surveys; current legislation or regulations that will be in effect for 2024, with which the designs would have to comply; capture the key specifications for the future vehicles; and outline requirements for a Proof of Concept vehicle design.
Lots and lots of data. It’s likely this data would be captured by different systems since the primary actors represent different lines of business (design research, marketing, safety engineering, legal, etc.). Some of this data would have to be integrated such that various alternatives and scenarios could be modeled.
Business Problems are Often Data Problems – As EDM professionals, we are more than familiar with this major business issue, but it can be challenging to describe the many impacts of data on business problems to executives. Inconsistent use of business terms, and lack of an organization-wide business glossary for highly shared data, negatively affects all business processes that rely on this data. Just to take a few examples: inability to uniquely identify a client / customer / patient, or count the number of same; incorrect billing or shipping addresses; financial reports that are inaccurate because the data comes from multiple sources and can’t be easily integrated; differences in the meaning of common terms (e.g. ‘head count’ may refer to the total number of FTEs in an organizational unit, or refer to employees available to be assigned to a task (those not on vacation, maternity leave, leave of absence, jury duty, US Reserves duty, etc.).
In a similar vein, the lack of availability of the right data at the right time may have a major impact on business decisions. If my business is professional services, and my legacy data warehouse produces 50 standard reports about utilization, but every request for alternatives or predictive analysis – such as ‘how many employees are working on Program A in countries 1, 2, and 3, what is their job grade level and utilization rate, and what will we look like next quarter?’ – requires manual integration or custom code, that’s a business problem. Decision-makers may have to manually juxtapose several standard reports or wait weeks for the O&M team to construct and run the complex query. A wrench in the works of efficient and timely decision-making.
In addition, if the condition of the data is poor, e.g. excessive duplicates, mis-matching codes, missing data elements, etc., it affects stakeholder confidence about business decisions.[2]
To raise the awareness of the importance of data quality, I’m a big fan of bearding the lion. I advise data management organizations to go directly to senior stakeholders and find out what their persistent issues are before developing a data quality strategy. The basic question here is: ‘How does the current condition of your data affect your business?’ In most organizations, you will surface many issues and aspirations, and you can propose a pilot project to demonstrate what some planning, effort, and toolsets can do to improve the data’s condition. Starting small with a highly visible data set can be a big boost to convince senior management that data quality efforts are worthwhile.
Lack of a clear scope – It can be challenging to define ‘enterprise data’ to definitively answer the question, “What data is within the scope of the EDM Function?” The general definition of ‘enterprise data’ is data that is very important to the organization, such that it benefits from enhanced management practices, e.g., mutually agreed upon definitions, an integration structure, authoritative data source designations, usage guidelines, etc. This question is often answered by physical scoping. For example, the data warehouse layer is enterprise data, legacy data stores are not. This approach seems simple but will miss some vitally important data sets. It’s more typical to employ a categorization and requirements-based approach, which may include the following considerations:
- Highly shared data – this category includes the data sets that are needed to execute more than one core business process, and which must be as timely and accurate as possible for all consuming business lines. Master data is an example – identification and core description data for highly shared entity types – such as Customers and Products.
- Required reporting data – this category includes the basic data elements, as well as derived data produced by calculations and aggregations, that are required for internal or external financial data or for external regulatory reporting. For highly regulated industries, an organization should determine its Critical Data Elements, an effort which is typically launched to fully describe data required for external reporting.
- Data essential for ‘line of production’ business processes – data vital for the core business processes of the organization, which is created and modified by a core business process, and consumed by more than one line of business, for example, Customer Accounts.
A notable accomplishment that some organizations have attained is to evolve towards the management of data by domains (aka, subject areas). Domains are described by the data set, rather than the system or repository in which it is stored. If the organization has an enterprise data model, the resulting domains can be quickly listed. Otherwise, the data sets resulting from applying the above categories should yield a rough boundary of what data is IN and what data is OUT. For example, data used by only one business line or by ancillary functions, such as a local database for tracking landscaping and lawn mowing tasks at the organization’s two locations, is not included.
Distributed culture and organization – Many organizations are comprised of distributed functions and business lines. This is particularly the case in older organizations that have evolved their structures organically over time to achieve specific business goals.
For example, a 100-year-old insurance company may have significant differences in its processes, terminology, and way of working from product line business unit. It may calculate its sales, risk posture, and future growth differently than other lines of business. Aggregate reports across product business lines are likely to be inaccurate and require manual workarounds to piece together. A critical yet conceptually simple question that an insurance company must answer is, “How much in overall reserves should we anticipate for the upcoming [time period]?” For example, for property insurance underwritten by the company in Houston, Miami, or New Orleans, what is the likely risk of a major hurricane this year, and what would the likely total claims amount be?
Another distribution factor is funding. Some organizations, including some very large ones with trillions of collars in assets, are structured such that funding is centered in the key business lines. In cases like this, one often encounters multiple information technology groups serving individual business lines, and a wide variety of technologies and products purchased to meet specific business requirements. Aside from excessive costs and lost efficiencies, software and tool vendors soon learn that they can go directly to line of business executives, leading to purchases that may not truly meet business requirements as they didn’t go through a rigorous selection process, and of course, a complex and difficult to manage technology stack.
Operationalized Data Governance – Continuingfrom the above paragraph, the establishment of an executive data governance council helps to convince executives to think organization-wide, or at least balance their needs with the needs of the entire organization.
If the organization does not have formalized data governance groups, there are major obstacles to: define the scope of enterprise data; gain agreements on shared key concepts / business terms; effectively integrate shared data; determine what metadata is required to build out the data knowledge base; develop consistent policies, processes and standards for data quality, etc., etc. Since governance is, by definition, collaborative decisions about shared data, it’s a case of ‘no hoof, no horse.’
If the organization is starting from ground zero on governance, it also is not likely to have achieved management of data by domains. It’s my observation that if starting from scratch, a bottom-up approach yields the quickest results: find a starting project important to multiple business lines, such as a Customer Master Data hub. Solicit line of business executives for some time from business data experts who will make up the ‘Data Working Group’ for the project. Train these experts in the tasks they will need to perform (e.g., writing a clear definition for a business term, creating a quality rule, etc.) and the types of decisions that they will need to make (e.g., what are the allowed values for the Customer Type Code and Customer Status).
Then start them to work with the implementation project team, allowing time for them to reach back to their business line and present draft terms and metadata from approval.
Data Management Organization – There are many organizations which simply have not yet been convinced that they need a centralized data management function and corresponding organization. The data management organization (DMO) is the backbone of managing data products and tools for the organization, and fulfills the role of the ‘shepherd’ of persistent data products and best practices – such as:
- Data management tools (e.g., data quality, data governance, metadata repository)
- Standards (data naming, data modeling, metadata, quality, classification, etc.)
- Policies and processes (e.g., data governance, creating and modifying business terms, data profiling, creating and changing metadata)
- Persistent products (e.g., the business glossary, the metadata repository, the list of critical data elements, the enterprise data model, etc.).
Without a DMO, there is no clear coordinator and permanent promoter organization for the EDM Strategy – and, as we can easily see from the above list, not a high likelihood of success in data management overall.When evaluating an organization against fundamental data management practices detailed in the Data Management Maturity (DMM) Model, the practices become more challenging to implement from Level 2 (Managed – program or business line scope of implementation) to Level 3 (Defined – organization-wide scope, recommended for all organizations as a target). I can say with high confidence that if an organization doesn’t plan to establish a DMO, it cannot achieve Level 3 practices. Therefore, an EDM Strategy is in effect, a bridge too far until a commitment is made to manage data at the enterprise level, that is, creating an organization dedicated to that principle. When recommending a slate of sequenced initiatives to these organizations, establishing a DMO is always the first order of business.
Lack of Organization-Wide Functions – indirectly, it is true that organizations which may not currently have a DMO or formal data governance may be better prepared to address these deficits if they have experience with other organizational functions that operate at the enterprise level. For example, it helps to have one or more of the following: an Enterprise Architecture group; a Business Architecture or Business Process Improvement (BPI) group; a well-established Internal Audit or Risk function, etc. If an organization is so distributed in it structure that it doesn’t staff for components that are intended to address the entire organization, it is less likely to be convinced of the fact that data is a strategic asset, that it should be managed as such, and that staff and resourcesare required to do so.
Having an Enterprise Architecture group is helpful because IT governance can extend to data governance, system owners can be extrapolated to data owners, and specifying a target data architecture is inherent in the mission of EA. Having a Business Architecture or BPI group is helpful because, after all, business functions and processes require data to execute, and a systematic approach to improve business processes will uncover corresponding data issues.
An enterprise Risk group is bound to run across data risk(s), and Internal Audit often develops findings that are in great part attributable to data. The message here is don’t despair, cultivate allies.
Lack of Executive Commitment – This is fundamentally due to a lack of understanding about the criticality of data, and frequently, a tendency to believe on faith that IT can and should solve everything data-related. Evidence can be seen in spotty funding, unwillingness to pay attention to quality issues, over-burdening IT resources with what should be a business responsibility (the data they create and manage), and reluctance to launch data governance and fund a DMO.
Since ‘executive opinion is your reality,’ you must set out to educate and change that opinion. The approach is a combination of fear – that is, pointing out the major business issues that prevent growth, leave the organization liable to financial, regulatory or reputational risk, etc. – and desire – that is, capturing the aspirations of the business and demonstrating how well-managed data is key to their realization.
A Solution Path – Is There a Quick Fix?
If some of
these obstacles are prevalent in your organization, you will benefit from
creative thinking and proactive engagement, in line with the tips suggested in
the obstacle descriptions. If many apply, you may also benefit from an
‘intervention’ – a time-boxed, multi-business line evaluation against the Data
Management Maturity Model, which was developed to bring disparate
organizational units together with common concepts, a common language, and a
consensus point of view about data management practices. The DMM is powerful
because it was designed to educate the lines of business about their inherent
responsibilities, practice by practice. Gaps are quickly seen; opportunities
for progress are quickly recognized; initiatives for rapid capability building
are pre-approved with the ‘voice of the customer.’ May be just what you need to
get the EDM ball rolling.
[1] In my classes, I compare EDM to Finance and Human Resources, as a permanent function that an organization will always need because it is always creating, managing, and using data. ‘Function,’ versus ‘program,’ is the preferred terminology because it implies permanence and non-discretionary, multi-year funding.
[2] There is a wealth of books, articles, webinars, and toolsets addressing data quality over the last 30 years. For the sake of word count, we won’t elaborate here, but you can view my TDAN.com columns How to Tackle a Data Quality Strategy and Accelerating Enterprise Data Quality for further information and solution paths.