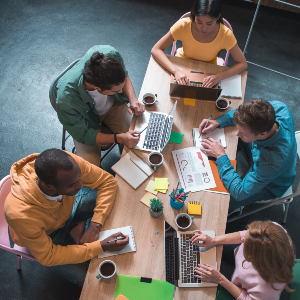
I had a great experience attending the MIT Chief Data Officer and Information Quality Symposium in Cambridge this July. It was truly enlightening to hear from so many experienced data leaders. This year, there were 2,855 registered attendees from 63 countries, including 1,218 Chief Data Officers. I always learn so much at these symposia. In addition to bringing me up to date on the latest buzz words, the speakers also gave me a sense the emerging trends and areas of focus as organizations strive to implement digital transformation.
From my perspective as an advocate for data and information quality, the emerging trends I gathered from this year’s symposium presentations held both good news and bad news. So, let’s start with the good news. Almost every presenter acknowledged that data quality played an important role in their digital transformation processes in some form or fashion. Though sometimes it was only one bullet point on one slide, even so, it was good to see it on the marquee. Even those presenting on machine learning admitted that model building efforts often needed help. They observed that their models tended to be better when both the training data and the input data have gone through quality assessment and remediation. This also included one of the newest data quality issues, bias detection and removal in the training and test data.
More focus on data quality at any level is a good thing. However, it reminds me of one of my favorite quips from Tom Redman who said, “Though it is universally praised, data sharing is the exception!” The same could be said for data quality. Every company claims they want clean and accurate data. Just read their data governance policies. They all have sage words to the effect, “…each business unit will document and implement data quality requirements and standards to ensure the data it manages is fit for purpose in its intended use…” But are they really devoting resources to make this happen?
So, what is the bad news? As my dad used to tell me, “Be careful not to pick the snake up from the wrong end son!” Loosely translated this meant that I should be careful to approach problems from the right direction. In my opinion, I find many organizations approach programs for data quality management from the wrong direction. As I discussed in my last column,t Data Speaks as Product, Deming, Juran, Crosby, and most other quality masters advocated starting with understanding customer needs and the characteristics of the product or service being built that produce value for the user of the product. These same principles apply to information product and services. Inspecting the final product or service to ensure that it conforms to these value characteristics is the process of quality control. Quality control is an essential part of the Deming PDCA cycle, ISO 8000-61 Standard, Six-Sigma, Juran Trilogy, and most other data quality management frameworks.
When this approach is applied to data quality programs, I like to call it “demand-side data quality management.” The approach is a chain starting with the value of the information product or service. Next, we determine the characteristics of the information product and service that drive its value. Once this is known, we can then determine the requirements for the source data and processes to enhance those characteristics. In other words, information value determines product characteristics. The product characteristics in turn inform the data quality requirements for the sources and processes that when met will have most impact on the information product’s value.
However, when I am asked to review data quality programs, I often find exactly the opposite. The entire operation is focused on profiling and scrubbing data, and usually quite vigorously. While this is not a bad thing in itself, it has bad consequences when it results in no one paying attention to the usability and value of the final information product or service being produced. This is apparent when there is little or no quality control or user experience feedback.
This approach to data quality is what I like to call “supply-side data quality management.” It seems to be driven by the “what could possibly go wrong theory,” The theory goes like this. If we put very clean data into highly tested and debugged processes, then everything will turn out okay, what could possibly go wrong? But without any understanding or assessment of how data cleansing is impacting the product value, we are just working in the dark. You could be spending time and energy fixing data quality issues in the source data that don’t impact the value of the information product or services you are building and you could be overlooking the quality issues that affect value the most.
The supply-side approach probably arises out of a common fallacy in propositional logic. From logic theory we know that if an implication is true, then it contrapositive will also be true, but the converse of the implication is not necessarily true. For those of us who merely gargled while passing by the fountain knowledge, I will elaborate in more detail.
If P Q (P implies Q) is true, then the contrapositive implication ~Q ~P (not Q implies not P) must also be true. On the other hand, the converse implication Q P (Q implies P) is not necessarily true. The implication of interest for this discussion is the statement “Garbage In, (implies) Garbage Out” also known as GIGO and which most of us are likely to agree is true. Framing this an information product context, we could state it as “Using Dirty Source Data (P), will produce (implies) a Low-Value Information Product (Q).” Then the contrapositive would be stated as “Having a High-Value Information Product (~Q), implies Clean Source Data must have been used (~P)” is also a true statement.
The problem is that we often wrongly assume that if P Q is true, then the converse Q P must also be true. The converse would be “Producing a Low-Value Information Product (Q), implies Dirty Source Data was used (P),” but as we all know, this is not always the case. It is entirely possible to build a low-value information product from very clean data. It could be an information product that is not fit for use for any number of reasons, for example, a report that nobody cares about or an information service that no one uses because there is a competing service that is less costly and easier to use.
Nevertheless, the converse of the GIGO is what drives the supply-side, can’t go wrong data management approach. In supply-side approach, the most important thing to be concerned about is whether the data going into the process is super clean, because if it is, it must follow that the information product coming will be good. Yeah, just pack it and ship it, no need to check it! Supply-side data quality management suffers from a lack of quality control over the final information products and services. It also lacks communication from the users of the information products and services back to the builders of the information products and services. Without this communication, you can’t formulate meaningful product requirements and consequently you have no basis for quality control.
I also see this same supply-side approach reflected in data governance implementations. You see it when the first phase is standing up a data catalog of sources instead of starting with a business glossary of information products and services. Most organizations beginning their data governance journey quickly find the key to success is limiting the initial scope. Even with crawlers and other robotic processes, standing up a comprehensive data catalog can be a daunting task. Wouldn’t it be better to start by populating your business glossary with the more limited inventory of your information products and services? Isn’t the whole purpose of digital transformation to realize more value you’re your data? If your information product and services are driving the value of your data, then shouldn’t your initial focus be there? Understanding the value-producing characteristics of your information products and services can help you determine the key data elements you need to catalog first and the key entities you need to master.
In any case, I hope you will give demand-side approach some thought as you review your current data quality management and data governance practices. And by the way, there is also a similar logical misunderstanding that often confuses people about the relationship between data validation and data accuracy, but let’s save that discussion for a future article.