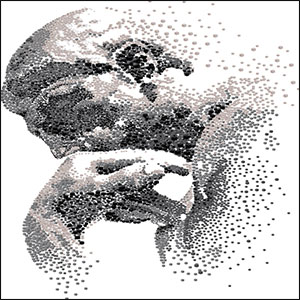
The term “data culture” is frequently used to describe a normative view about how an organization functions (or more precisely, should function) with respect to its data. The term is not particularly well defined, and the notions held about this term can vary significantly. Many data management professionals think of an organization’s data culture as that “je nais sais quoi” quality (think of a glowing halo representing virtue), and I surmise that if they were surveyed about what a strong data culture is, the consensus answer might be: “I know it when I see it.”
Organizations frequently state that they are committed to developing a “data-driven” culture, but the emphasis here tends to be their intention for decision-making and analytic activities to align with factual and verifiable information (data). A very worthy goal, a valuable subset of what makes up the data culture. In addition, internal data evangelists may wave their hands and declaim, “We need to develop a data culture!” To generalize, the vision of what organizational practices and behaviors constitute a broad-based, successful data culture seems to be subject to a range of individual interpretations.
I submit that we need to craft a set of requirements that can be agreed upon and accepted by a broad range of industry professionals. If we don’t attempt this clarification effort, we, the data management industry, are left with considerable ambiguity — the equivalent of “I don’t know about art, but I know what I like.”
This topic has arisen for me recently in the context of the EDM Council’s DCAM 3.0 Working Group.[1] One of the topic focus areas is data culture. As of this writing, the group is currently in the early-to-mid stages of our analysis. We are discussing data culture from several points of view: what new content should be added to the DCAM, where would it fall within the current framework structure, what changes we propose to that structure, what modifications should be made to existing content, how the new/modified content would be assessed, and so on.[2]
Once these determinations are well-articulated, agreed to by the group, and then approved by the Council, content-aligned groups will spin off to tackle the writing assignments. (Note: If your organization is currently using DCAM 2.0 as your DM guiding framework, rest easy, development of 3.0 will take a while, and yes, modifications will be mapped to 2.0.)
The data culture sub-group is currently at the stage of applying our initial topic lists (relevant factors which we think collectively describe aspects of a data culture), and synthesizing key points into evidenced activities and behaviors. That is, requirements (in DCAM terms, Sub-Capabilities) for what an organization would need to demonstrate along the axis of data culture.
One can begin decomposing data culture from a high-level vision, which summarizes what the organization has accomplished when it can feel confident in asserting that, “We have a strong data culture.” One can also compile a collection of activities and behaviors that demonstrate a developed data culture, and then categorize them and parse them into the DCAM. Or, one can apply a combination of the two approaches, which is the path the working group has followed.
The working definition[3] posited to date includes a summary description of a strong data culture: “A strong data culture promotes data-driven decision-making, data transparency, and the alignment of data and analytics to business objectives. It prioritizes strategic data use and encourages sharing and collaboration around data.”
After extensive discussions, with participants offering numerous characteristics that they thought were important aspects of data culture, our group leader drafted a categorization of six general themes that collectively address aspects of data culture. Below, are some highlights of the group’s brainstorming sessions.
- Data literacy – includes foundational education about concepts and principles, e.g., data for all, data criticality, understanding the data, understanding data value, common terminology, minimizing redundancy, etc.
- Data culture characteristics – includes shared values, data sharing (as appropriate), business engagement, executive advocacy, data-driven business processes, staff role scope is universal (everyone with a data-related role is on board), shared responsibilities for improving data quality, etc.
- Data skills – includes numeracy, scientific method, data utilization, data management competencies (from conceptual / project approaches to specific training as needed), etc.
- Data governance – formalized collaboration, formal data accountability (RACI), designated data owners, governance metrics, policies, and standards, etc.
- Communication – informing all relevant parts of the organization, bi-directional loops (from the data management program to stakeholders, from governance to the data management program, from governance representatives to their business lines / domains), communications strategy/plans, channels, frequency, origination levels, executive encouragement, etc.
- Change management – effective adoption and rollout processes (for policies, processes, and standards), mentoring, adoption, and compliance metrics, etc.
General characteristics, which would provide observable evidence of a successful data culture were expressed as:
- Embeddedness and universality across the organization – all organizational stakeholders with responsibilities for enterprise data are performing the practices and behaviors.
- Principles defined and accepted – organizational goals for managing data, such as “data first” or “minimize redundancy.”[4]
- Data transparency – easily available and comprehensive information about the data.
- Data leadership – executive advocacy, encouragement, and recognition for staff.
You can observe that the proposed characteristics do tend to reinforce each other. In an attempt to categorize these topics in a structure that can then be parsed into capabilities suitable for a data management assessment model, the group is currently discussing how to define and assess each proposed requirement.
If relevant new topics were added to the DCAM, in combination with enhancing those already present such as Component 6.0 Data Governance, it would be possible to get a reasonably accurate, compiled view of the organization’s current state with respect to developing a robust data culture. The new (or existing, or enhanced) Capabilities and Sub-Capabilities would be “tagged” in the scoring mechanism. Why do I use the term “tagged?” Because evidence of a well-developed data culture will be found within multiple Components and Capabilities in the DCAM 3.0. This is as it should be, as data culture is a lens that can be applied to the organization (or business line, or horizontal shared repository) as a whole, as well as to defined components of the data management program.
Identifying and specifying these requirements and tagging them would make it possible to aggregate the scorable elements that collectively make up the data culture, and come up with a numerical Data Culture Index score, as an enhancement to the DCAM’s measurement dimension. In short, The DCAM would help organizations to make the (somewhat intangible) notion of data culture more tangible and measurable.
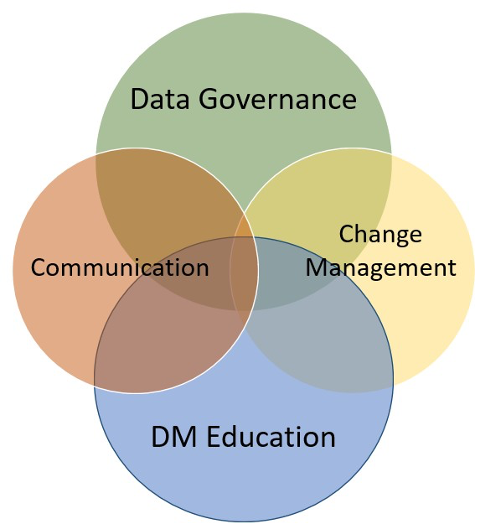
As befitting the name of this column “Data Professional Introspective,” I’m sharing my current thinking with you. As an informal homework assignment between meetings, I set out to abstract and decompose data culture characteristics, in an attempt to encompass concepts and evidence into a more systematic package. For simplicity, I employed a Venn diagram to visually indicate dependencies and overlaps — since many aspects of a data culture are related, and some are dependent on others.
So, let’s unpack this diagram, starting with …
Data Management Education
A data culture must be developed, and it must grow and evolve. The foundation for that is staff education, and organizations need to define a strategy for an educational program, with the rationale for what their staff resources need to know, and what roles are appropriate for what levels of data-related education and skills.
The responsibility for leading this effort should rest with the Data Management Organization (DMO), incorporating ideas and input from Data Governance. For instance, every organization should develop (or purchase) a short course on introductory data principles and concepts that should become familiar to everyone who touches data in their job function — the bedrock of broad-based data literacy.
Many organizations have developed such courses aimed at both existing and new employees. One excellent example is the program developed for the State of Arizona. Jeff Wolkove, the State Data Management Architect, developed a 20-minute data literacy course (“Introduction to Data Stewardship”) for every individual with a data-related role (estimated at 22,000 people across 100 agencies), which has been taken by thousands of State employees to date.[5]
Organizations are advised to develop a comprehensive role-based education program aligned to data management roles and master RACI, according to a Data Management Education strategy. The strategy would present the rationale for the program, and assign staff data roles to a suite of courses appropriate for their role. Once the strategy is approved by the executive governance body, and the course content outlined, the organization creates (or purchases) courses and offers them.
For instance, a data literacy course for everyone, one (or more) data stewards’ courses for those representing business or technical organizational units, one (or more) courses for data domain owners, etc. In the case of Arizona, there are three successive courses; data owners must take all three. It is further advised that the organization develop an education policy, and measure progress, as in the example of Arizona’s Intro course (target audience 22,000, 7,000+ class completions to date (32%)).
Both executive advocacy and organizational collaboration are critical for the success of a data management education program. The organization may add tools training to the role-based scheme, as appropriate, e.g., data stewards should learn how to use the governance workflow platform, business users need to learn how to use business intelligence software for self-service reporting. In addition, the program should include conceptual courses — coupled with how-to instruction — for data profiling, data requirements, metadata management, data provider management, and other data management tasks and skills.
Data Governance
Data Governance is essentially collaboration — making mutual decisions about data important to the organization. It is an essential business function, a critical component of the data management program, just as important as the centralized leadership function of the DMO. The business owns the data it creates and manages. The essential foundation for effective data governance is development of a well-organized structure and defining roles; and the biggest challenge is fostering engagement across the organization.
I’m not going to reinvent the wheel here, since data governance is the subject of roughly 50% of data management publications and frameworks. I would, however, like to comment on the industry emphasis. Many publications emphasize the aspect of assigning authorities (Accountability and Responsibility in the RACI matrix) and defining processes that enforce exercising control over the data through its lifecycle. The organization’s governance framework, policies, and processes must succeed in analysis and designation of these functions, both across stakeholder groups and for named roles or individuals who participate. Decision and escalation processes need to be defined and implemented. This is vital for the success of the data management program, and the DCAM Component 6.0 Data Governance fully addresses the practices that constitute fully implemented control functions across the organization.
However, the value of data governance, and its essential contribution to the data culture, goes beyond the controlling function. In a robust data culture, there must also be evidence of broad participation in two additional areas of collaboration:
- Building – Activities that support enhanced understanding of the data assets, and development of persistent data management products, for instance, developing harmonized and approved shared business terms, defining shared entity types and attributes to a data warehouse or master data hub, and analyzing and arriving at consensus on key decisions affecting shared data initiatives. For example, input to the metadata strategy and determining priorities for a population of the data catalog.
- Sustaining – The sustaining function requires that the governance structure and approach establish ongoing responsibilities for providing input to and approving data management policies and processes on behalf of the data domain or business line they represent. In addition, the sustaining function of governance focuses on business-led assessment of data quality requirements, standards, and controls. A sample of governance tasks within this function include:
- Ongoing decisions about business terms (defining, adding, and changing).
- Developing and approving metadata properties and priorities.
- Developing targets, thresholds, and quality rules for shared data.
- Determining linkages across data sets needed by multiple business areas.
- Proposing and approving additions or changes in shared data stores.
- Engaging in data quality profiling and cleansing efforts.
- Engaging in data standardization efforts, such as semantic models and cross-industry practices and standards.
Effective data governance is dependent on role-based education, requisite skills, and adoption of an ‘altruistic posture’ with respect to shared data, that has multiple downstream consumers. It requires effective communication and engagement in adoption and change management activities. To motivate active, enthusiastic data governance, business executives need to encourage, recognize, and reward governance participation. Measures and metrics should be developed to evaluate the degree of participation from business staff in these activities, and reported regularly to the executive governance body.
Data Management Communications (DMC)
DMC is an important sustaining function for the evolution of the data culture. Since the organization needs to manage its data forever, and the data management program is thus required forever, it’s important to inform stakeholders, showcase progress, and encourage engagement. There are multiple bi-directional communications avenues between different stakeholder groups within the data management program. At a minimum, the following buckets:
- Communication between the DMO and the business lines / data domains – Program level metrics, announcements of new policies, processes, and standards, etc.
- Communication between governance groups, such as information provided by designated data stewards to the business lines / data domains they represent, and vice versa – Decisions of the governance group, requests to review and approve policies and processes, updates on persistent data products (e.g., new domain populated in the data catalog), etc.
- Communication between the DMO and governance groups –Agendas for the executive governance body, action item tracking, reviews, and approvals outstanding, metrics on data management projects, status of escalated issues, etc.
The organization is advised to charge the DMO with leading the collaborative development effort for a data management communications strategy. The strategy should define data management communications goals and objectives, describing communication types, and identifying stakeholders and audience groups. It should include a description of channels (e.g., email, web portal, virtual or face-to-face meetings, quarterly all-hands, etc.) and what communication types would typically be accessed through those channels. By communication type, the strategy should indicate the typical frequency of distribution, and also define an initial set of measures and metrics and indicate to whom and how frequently they are reported.
A thoughtful communications strategy will help to foster business support, motivate participants to become engaged with governance groups, and ensure the success of new policies, processes, standards, and data transformation initiatives. It’s important to solicit and incorporate frequent feedback from audience groups to improve effectiveness, for instance, feedback on data management sessions introducing policies, processes, and standards, feedback on the frequency of informational emails, etc. Leveraging automated features will also help develop measures and metrics. For example, capturing emails opened, tracking downloads of new DMO publications, etc.
Change Management
Change management is a discipline that high-functioning organizations employ as a matter of course when undertaking re-organizations or mergers, introducing new core systems such as case tracking systems or customer relationship management systems, and moving from one office tools platform to another, e.g., Microsoft to Google or vice versa. It is advised to apply change management best practices to the data management program. Once again, the DMO should lead these efforts, with significant input from data governance and those who will be affected by new policies, processes, standards, and data management software tools.
It is recommended that organizations consider developing a change management strategy aligned with the data management strategy and roadmap. It will lay the foundation for specific corresponding plans, to assist staff in learning the role they have in new or modified practices. A well-organized change management approach will enhance the likelihood of success for the data management program.
Change management depends on an effective communications program (addressed above), as well as foundational knowledge on the part of the audience, established by education. It requires participation from leadership, analysis of the firm’s culture and consideration of the human factors related to change — challenge, uncertainty, anxiety, loss of control, competitiveness, opportunity — and learning how to execute work in the ‘new’ environment.
There is a marketing component to convey the benefits and advantages of changes, as change is also rewarding and a learning and growth opportunity. It can be managed successfully through planning, listening, communicating, using the right tools, and an emphasis on the benefits of the change for the individuals and the firm. The business reason for the change and the resulting benefits need to be clearly communicated.
The organization’s change management plans should consider what needs to be accomplished to realize meaningful improvements from the proposed change:
- Seek input from those affected by the change, and determine what will be different and what the new requirements are.
- Describe a clear vision of the change — e.g., a new data quality policy.
- Identify those affected by the change, including roles upstream and downstream from the change — e.g., data domain owners, technical data stewards.
- Different audiences may require tailored materials / presentations.
- Determine the education and/or skills foundation that the varying audiences should complete prior to rollout.
- Secure adequate funding and time commitments — e.g., virtual meetings, budget for workbooks, modifications to web portal, etc.
- Select effective change leaders, including those now responsible for current processes
- Executive advocacy for the change should be encouraged, and should provide positive recognition for each achievement of moving to the new policy or process.
- Capture measurements and produce metrics as an integral aspect of the change management rollout, for example, number of attendees at brown bag events, number of downloads for new documentation, post-rollout survey responses, etc.
In summary, the framework I’ve outlined builds upon the practices described within these four functions to establish and maintain a robust data culture in any organization. As I mentioned above, this framework is under active discussion and review, so I’m very interested in your comments. Feel free to email me with your comments, and Happy New Year!
[1] Led by Rob Wentz, EDMC — a consummate DM professional who has led numerous DCAM Assessments
[2] Participation in the DCAM 3.0 working group is my third time around wrestling with a DM framework. The original Content Model starting in 2011, which became the DMM and the DCAM, and the development of the DMM, published in 2014. I wasn’t on the original DCAM team, but have joined the party — a labor of love for all involved.
[3] Emphasis on “working definition.” This is subject to iterative refinement.
[4] Aka, Sprint Zero in an agile delivery environment, data models reflect functional requirements, etc.
[5] Earning high satisfaction scores from students, by the way.