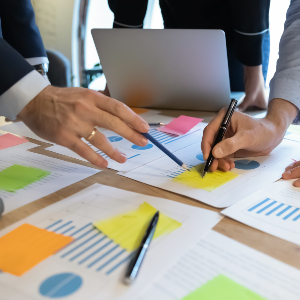
Data professionals often talk about the importance of managing data and information as organizational assets, but what does this mean? What is the actual business value of data and information? How can this value be measured? How do we manage data and information as assets? These are some of the questions that I intend to address in this series of articles.
In the first article, we talked about the different ways that data and information can be used to create business value. In the second article, we identified data as a special type of circulating asset and discussed its particular characteristics. The next question we need to address is: How exactly should data be managed so as to maximize its value?
How Do We Manage Data Assets?
Data and information are organizational assets, and as such, they need to be managed at an organizational level. Each business unit can’t have its own “truth” — that would be like each state or province in a country having its own currency. But how do we manage them in a way that creates value?
What often happens in organizations is that businesspeople collect and hoard data from wherever they can get it, in Excel spreadsheets and Access databases, like squirrels gathering nuts for the winter. They manipulate and filter the data in various unknown ways to suit their individual purposes, then they often share this data across the organization where it is used in ways that may be inappropriate, or downright dangerous. Over time, this disparate and low-quality data can cripple an organization’s ability to make correct decisions, or to respond effectively to new business challenges. I sometimes use the analogy of moles, whose activities can cause much destruction to people’s lawns and gardens. The moles don’t do this intentionally; they’re only trying to build homes and feed their families. But the ways in which they try to meet their own needs can produce devastating results!
In my book on business intelligence,[i] I apply two fundamental laws of economics to the management of data and information. Gresham’s Law, familiar to most people, states that bad currencies eventually drive good currencies out of circulation. But there’s a corollary to Gresham’s Law, called Thier’s Law, which says that Gresham’s Law applies only to “fiat currencies,” that is, in cases where the government (or some similar authority) decrees that both currencies have the same value. For example, if the government decrees that a copper-and-nickel based coin with a silver coating has the same value as a solid silver coin, people will hoard the more valuable coin and keep the less valuable coin in circulation. The “bad money” would drive the “good money” out of circulation. But if people were allowed to place their own valuation on the coins, they would prefer to trade with the more valuable coin, and thus the “good money” would drive the “bad money” out of circulation.
Why am I telling you this? Because Gresham’s Law and Thier’s Law apply to Data and Information, as well as to currency! If bad data and bad information are regarded as no better or no worse than good data and good information, then disinformation will eventually win out (if for no other reason than it’s easier, faster, and cheaper to get and use bad data). But if good data and good information are regarded as more valuable (and are just as easy to get and use), then good information will drive out disinformation.
What this means is that we need to create data and information assets that are regarded as more valuable and useful than the “bad currency” of locally controlled Excel and Access data and make these assets quickly and easily available across the organization.
So, the question is, how do we create a “good currency” of high-quality, business-relevant, reusable data that can drive the “bad currency” of Excel and Access data out of circulation (or at least keep it under control)? Here are a few ideas:
First, define (i.e., model) data assets at as high a level in the organization as possible. Identify which data entities and attributes and which business rules pertain to the organization as a whole, which are canonical (that is, they span multiple business domains), and which pertain only to certain business domains or subdomains. There is a current approach to business intelligence called data mesh, in which all data is defined at the domain (i.e., business subject area) level, and the results of analytics (called data products) are created and published at that level. The problem with this approach is that much of an organization’s data spans multiple business domains and needs to be defined consistently across the organization in order to be useful.[ii] Similarly, it needs to be known whether the results of analytics are applicable across the entire organization, or only to a particular division or business unit.
Second, data needs to be managed for quality, timeliness, consistency, reusability, and business relevance. This may mean, for example, managing enterprise-level data assets in a master data management (MDM) catalog and publishing this data across the organization. It may also involve maintaining a common repository (e.g., an enterprise data warehouse or something similar) where organizational data assets and data products can be managed for consumption and reuse.
Decades ago, an economist named Garrett Hardin published an essay called “The Tragedy of the Commons,” showing what happens to assets that anybody can use, but that nobody manages or maintains. Those assets become corrupted and eventually fall into disrepair and disuse.
Third, make sure there is a formal process for creating, maintaining, using, and publishing data and information assets. This is called data governance and is essentially a set of rules established by the business governing how people should behave with respect to data and information (remember what I said earlier about asset management!). Data governance can be effectively implemented at the business domain level, with guidance and supervision from higher levels of the business. This fits in well with the data mesh approach,[iii] and Robert Seiner’s “non-invasive” approach to data governance.[iv]
Fourth, don’t forget about metadata! The purpose of metadata is not simply to describe data and information assets, but rather to proactively answer questions that consumers might have about them. Where did this data come from? How up to date is it? How trustworthy is it? What business process(es) created it? What business process(es) use it? What transformations or filtering have been applied to this data, and why? What is the business meaning of this data? What is its value to the business? What business purposes can this data be used for? What can’t this data be used for? Use metadata to maintain the transparency of data and information assets across the organization and ensure that these assets can be easily found, used, and trusted.
Fifth, make sure that data and information assets are published and accessible across the organization, and make sure that people know where and how to find them. Educate users on where and how to find good data, how to tell good data from bad data, how to avoid common data usage errors, how to determine when the results of analyses may be incomplete or incorrect, and how to report data errors and problems for quick resolution. Also, make sure that less-trustworthy copies of the data are identified and deprecated.
Finally, take an iterative (i.e., agile) approach to data management and BI. Don’t try to boil the entire ocean at one time. Take direction from the business as to which data and information assets are most important to the organization and create a workable process that can be executed iteratively to improve both the data and the data governance process over time.
In the next article, we will explore the topic of how the business value of data and information can be measured.
Image used under license from Shutterstock
[i] Burns, Larry. Growing Business Intelligence (Technics Publications, 2016).
[ii] See my TDAN.com series of articles on domain-driven development and microservices. The link to the fourth article is shown below; links to the other three articles are in the opening paragraphs.
[iii] Burns, Larry. “Domain-Driven Development, Part 4: Data Mesh and Data as a Product” TDAN.com, September 21, 2022. tdan.com/domain-driven-development-part-4/29883
[iv] Seiner, Robert S. Non-Invasive Data Governance (Technics Publications, 2014).